Interviews
Dave Boundy is the CTO at Innowatts – Interview Series
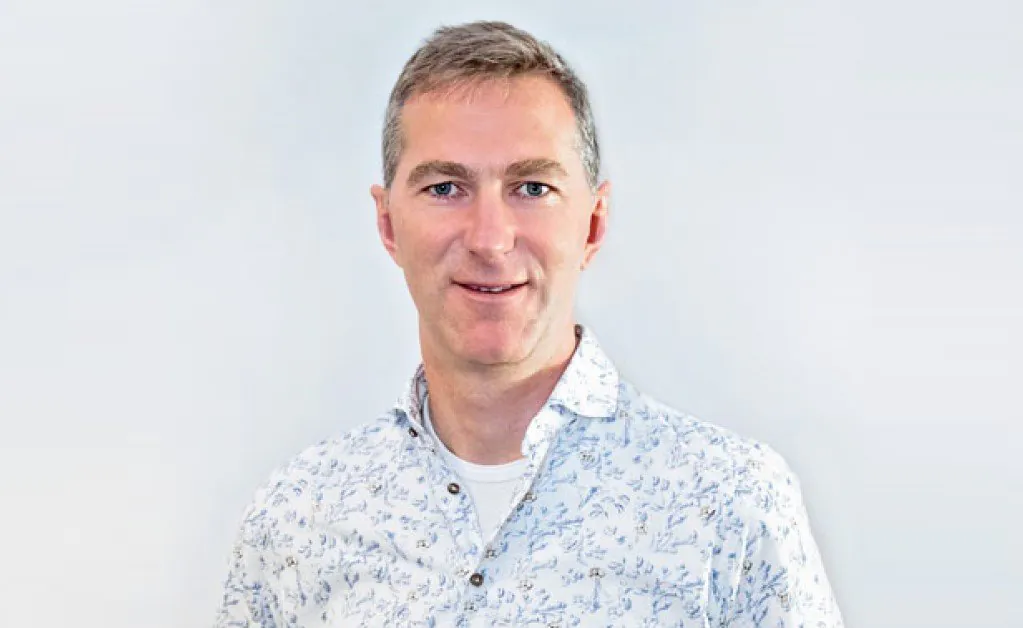
Dave Boundy is the CTO at Innowatts, a leader in AI data analytics. The SaaS platform provides companies with the data needed to be more predictive, proactive and connected to their customers and ratepayers, helping them better manage risk, improve profitability, maintain grid reliability and anticipate sustainability trends.
David has over 20 years IoT and Energy industry experience. His career has ranged from enterprise IT, product development, research & innovation and new business start-ups. Prior to his current role, he established and led Intel’s Global Energy Solutions business, delivering machine learning and artificial intelligence solutions to customers worldwide. David holds several patents and sits on a number of advisory boards.
Could you describe what Innowatts is?
Innowatts is a new energy company focused on improving our customers’ gross margins while driving increased adoption of renewables. We do so by analyzing large amounts of data to unlock actionable insights. While we are a B2B company, we provide both B2B and B2C services through our customers to their end users. We take meter-level data from a home or business, or within the network, and we combine that with many other data sets including socioeconomic data, CRM data, and risk management data.
Our AI based analytics engine delivers insights ranging from consumer-level forecasting all the way to disaggregating current and future energy usage. Through this, we can identify potential products suited to any consumer. We also provide retail customers with services for example identification of the optimal tariff model to maximize their gross margins.
Finally, we support network operators and utilities to help them plan for the future. It’s essential that they understand where changes are happening so they can optimize assets while reducing prices for consumers.
What type of machine learning technologies are used at Innowatts?
Essentially, we’re helping machines to think in more human ways, while also allowing them to scale and deal with very large datasets. We have more than 43 million meters under management, and we’re dealing with billions of data points every hour across multiple geographies and multiple customers. In the supervised space, we use a variety of models including linear regression, decision trees etc; in the unsupervised space, examples include ensemble models, neural networks and random forests.
Our use of a variety of models is central to the strength of our product — we can identify the right model for any customer in any given situation. We can actually boil it down to the meter level. That’s what really positions us as a leader in this space.
Why is energy monitoring important for energy retailers?
The cost to acquire a customer is often two to three times the margin that a retailer makes on that customer in a year. That means it takes two to three years to earn back your customer acquisition costs, so it’s really important to retain every customer.
We help retailers to achieve that by giving them the ability to differentiate. We provide forecasts and the ability to define and develop time-of-use tariffs to tailor their product to their customers. We can also provide risk management and pricing platforms, along with the ability to identify the best consumers in each territory. We also identify which customers are best suited to particular products. That all adds up to a more compelling and cost-effective service for customers, boosting both acquisition and retention efforts.
What are some of the key insights that can be gained from studying energy consumption?
Imagine that you recently bought an electric vehicle. Our models can detect that, allowing your electricity provider to automatically send our bespoke communications and targeted services applicable to your status as a new EV owner — perhaps a different tariff, a new insurance product, or a servicing product.
Similarly, we can identify and proactively notify you, based on your energy usage over the last 15 days, that we’re projecting your consumption during the next 30 days to be much higher than normal for a particular reason. We can then work with you to take preemptive action to avoid a bill shock. These are just a few examples of the kind of engagements that we can deliver.
Our forecasting capabilities are also extremely powerful. Recent events in Texas made forecasting very difficult, because it was a completely novel scenario. But our forecasts were 20% to 40% more accurate than our competitors’, so our customers were far better prepared for the disruption. By using our forecasts as part of their hedging strategy, they were far better positioned as energy prices soared to $9,000 per megawatt hour. The savings for them have been significant. The fact that our models adapt quickly to changing situations also enables our customers to forecast and bid effectively in highly volatile situations, even amid rolling blackouts.
This level of monitoring and interpreting energy data is increasingly important. Many retailers will be going out of business, and the large retailers have hedged their requirements in the market up to a year, maybe longer. Depending on their strategy and risk profile, they may have acquired 50% of the required energy they needed in the last week. They would have tried to increase that percentage as the risk profile and energy price was increasing. They would have hedged more, but ultimately, they would have been going into the real-time market trying to purchase power. They were having to do that at the average price for the last year, which has been about $25 a megawatt hour. Now going into the market, they’re trying to buy at $9,000 per megawatt hour. So the cost for the retailer is huge, that’s their year’s profits gone in an afternoon.
What are some ways this information can be used to influence usage for greener energy?
Historically, the grid has been demand-led — when demand increased, operators would build more power plants, put in more cables, burn more fuel. We’re now in a transition phase where we’re moving to a supply-led grid. As we move to renewables, we need to be able to manage the supply side. In the new world, supply arrives whenever the wind blows or the sun shines. We’re also going to see a huge increase in demand stemming from the electrification of transport and heat.
What we do for our customers is analyze how consumers use energy. We start off by looking at how much of their load is what we call “base load.” There are some things that can be done to incentivize people to reduce that, but by and large, it’s fixed. We can identify those who are susceptible to weather conditions, we can identify homes that could do with thermal interventions — insulation or putting in a thermostat.
But we can also identify time-sensitive loads, which is an indicator of behavior. Without getting too invasive, we can see home-based behaviors. For example, you can advise a customer that they might want to consider cooling or heating their home at a different time to make it more comfortable, save money, or maximize their use of green energy.
We can also use proactive, demand-side management strategies to align consumption to the availability of renewable energy without negatively impacting the user’s experience. For example, you might routinely plug in your vehicle at 7 p.m. and unplug it at 7 a.m. We can send a demand-side management signal to the charge points to determine the hours when your vehicle actually charges, in order to optimize the use of renewables.
In 2020, Innowatts announced that it had outperformed five other energy forecasters on most measures in an independent trial. How valuable is it to be able to forecast with such a high level of accuracy?
The situation in Texas showed the cost of inaccurate forecasting. If a retailer is looking at a potential half-billion dollar loss, then our forecasts — which are 20% to 40% more accurate than the competition — could conservatively save them well over $100 million. That’s an extreme situation, but our granular approach means customers are coming out ahead year-round, even without extreme volatility.
For example, we’re supporting a provider in Europe with both commercial and industrial customers. They’ve been trying for years to get proactive notifications of when their customers are going to take actions — when they’re going to increase or decrease their power — but they haven’t been successful. We’ve been able to forecast for them at 15-minute intervals for each of their commercial and industrial customers, allowing them to anticipate load changes and make smarter purchasing decisions at a highly granular level — and that means they’re able to save significant money.
What is the future of data science and machine learning in this sector?
As I mentioned, we’re dealing with very large datasets. As we get more data, and more granular data, we’ll be able to deduce and infer more information — and as computer power increases, we’ll be able to process that data more quickly to deliver powerful insights. The mathematical principles don’t change, but our growing ability to apply complex math at scale will make these capabilities increasingly effective, impactful, and affordable over the next few years. Ultimately this will support the drive towards a decarbonised grid.
Thank you for the great interview, readers who wish to learn more should visit Innowatts.
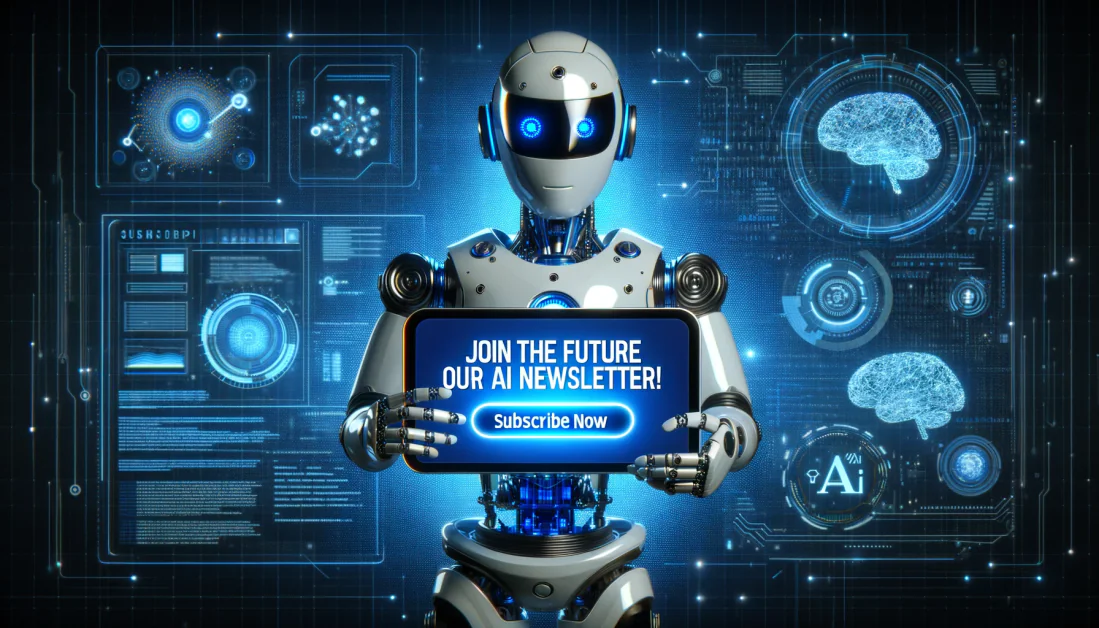
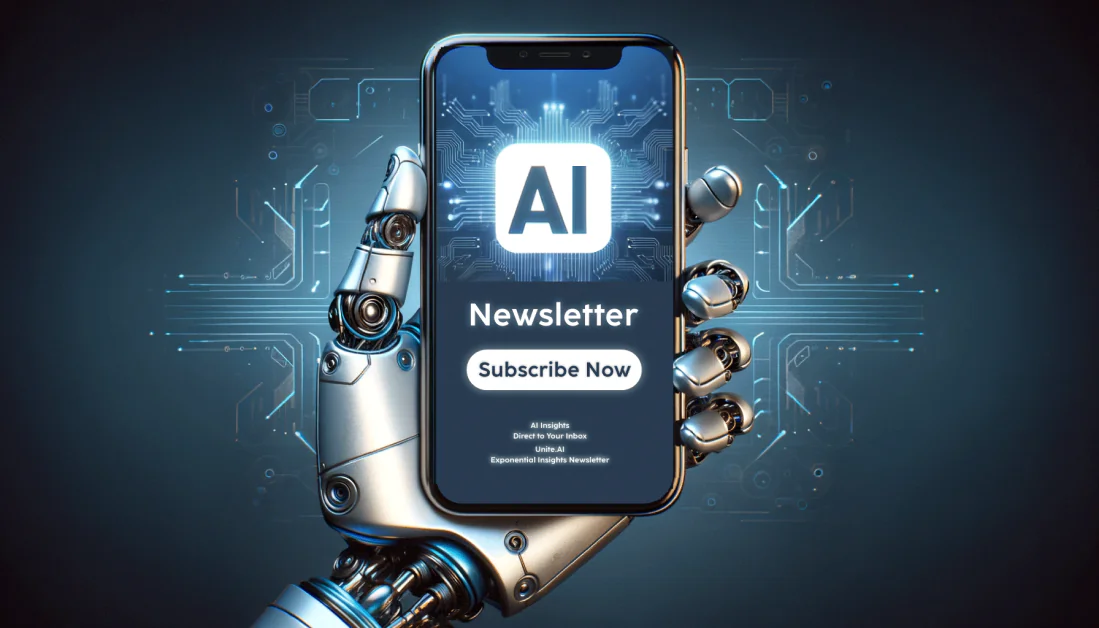