Interviews
Benjamin Sexson, CEO Monogram Orthopaedics – Interview Series
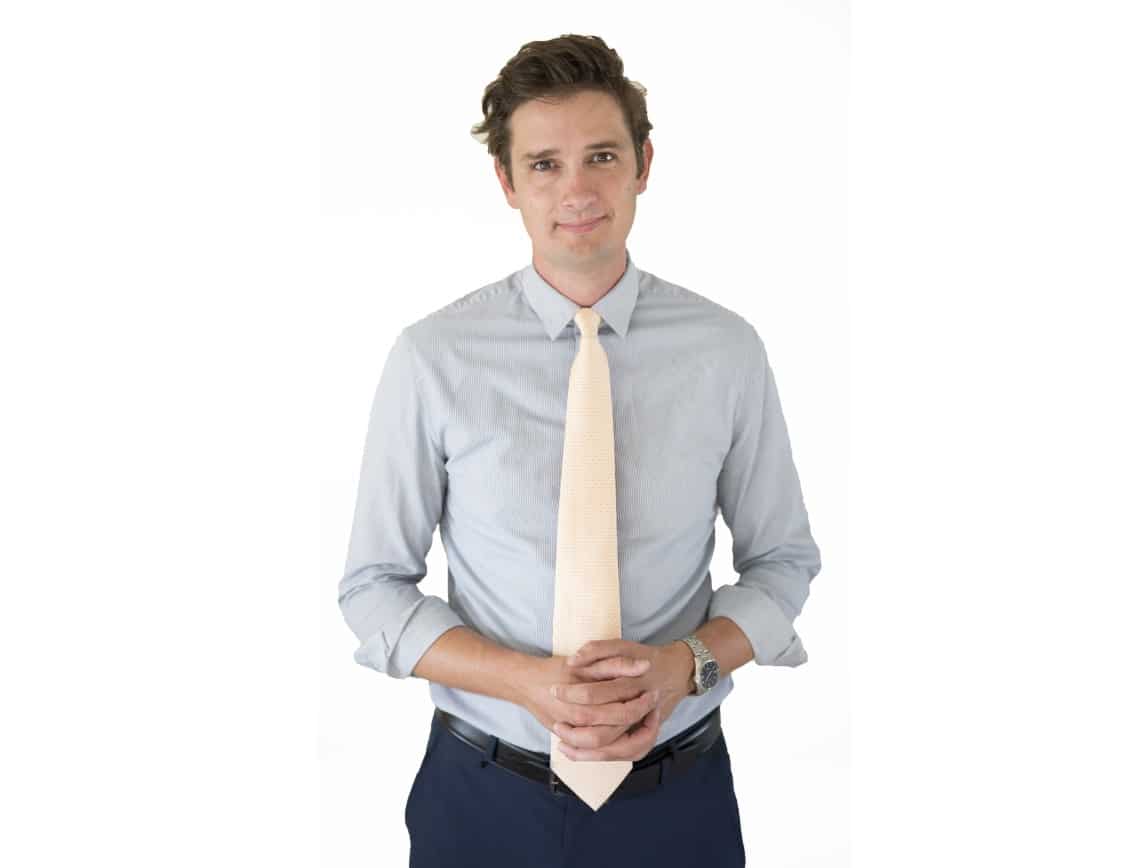
Benjamin Sexson is the CEO of Monogram Orthopedics. Prior to joining Monogram, Mr. Sexson served as the Director of Business Development at Pro-Dex, an OEM manufacturer of Orthopedic Robotic End-Effectors. In his tenure at Pro-Dex, Mr. Sexson was responsible for the development, management, and launch of a proprietary product solution, helping to negate a distribution agreement with a major strategic partner.
Could you explain the mission statement of Monogram Orthopaedics?
Our Mission is to make orthopaedics personal. The current standard of care is highly impersonal. In crude terms, patients are permanently and irreversibly amputating arthritic bone to have it replaced with an off-the-shelf generic implant that, in non-clinical terms, gets “hammered” into place. We quite literally are replacing joints with implants that are guaranteed not to fit perfectly. We are working to mitigate the risks of arthroplasty with technology that drives personalization.
What type of scans are necessary in order to fully design the 3D molding for the knee or hip replacement?
We are utilizing computed tomography scans (also CT scans). Optimally the CT examination is performed at 140 kV and 300 mA with slice thicknesses of 0.625mm in regions of interest and thicknesses of 3-5mm in areas where we aren't reconstructing anatomy with an implant.
How is machine learning used to parse the data from these scans?
Bone is a composite. It consists of compact bone at the periphery, spongy bone, and bone marrow. Our implants are designed to maximize contact with the inner cortical wall (inner surface of the compacted bone at the periphery) to improve initial stability.
The primary purpose of the machine learning algorithms is to segment bone (inner and outer cortex) from the CT scans as well as to identify the critical anatomical landmarks that inform the implant design algorithms.
Where does the 3D printing take place?
The FDA mandates highly rigorous quality standards. Initially, we will be using an ISO 13485 contract manufacturer. As we scale, we will vertically integrate.
Monogram will leverage the kinematic redundancy of robotics to allow for intraoperative obstacle avoidance and real-time tissue tracking. Will this decrease the risks of surgery complications such as blood clots or infection?
Our innovation starts with the implants – we are driving improved fixation in a smaller form factor. Infections with implants can be especially insidious and a leading cause of revision. Generally, once a thin, slimy film of bacteria commonly referred to as biofilm adheres to the implant surface, the infection is irreversible, and the implant removal is required. By reducing the size of our implants, we reserve bone stock for future revision. Furthermore, we hypothesize that our increased cortical contact will facilitate more even and proximal loading of the femur, mitigating the risk of stress shielding.
Notably, Monogram has commissioned research in collaboration with a pharmaceutical company to explore a proprietary coating with very high adherence that may reduce the risks of infection without being cytotoxic.
Will this speed the healing time for patients?
It is unlikely to reduce the healing time for patients, but it is likely to mitigate the risks of loosening, fracture, and poor placement. Improved initial fixation can lessen pain from implant migration. We also believe we will preserve more bone stock in the adverse event a revision is needed. Our implants rely on natural, long term fixation, which is especially favorable for younger active patients.
You recently chose to fundraise a Series A via the SeedInvest crowdfunding platform. What inspired you to crowdfund versus traditional VC funding?
Monogram needs a significant amount of capital to navigate the FDA approval process and commercialize our technology. The problem with traditional VC financing is that a financing round for large deals generally needs to be fully syndicated before a company has access to capital. With SeedInvest, it's more like a shelf-offering. Once we hit our escrow target of $2.75M, we have access to those funds immediately, allowing us to hire talent and continue our development efforts as we fundraise. Furthermore, we are laying the groundwork to go public down the road, so this is highly strategic for the long term.
Monogram is in the process of onboarding a new VP of Engineering and Director of Implant Engineering. The next steps are finalizing discussions we are having with a strategic partner for licensing the generic components of our design (for example, the tibial locking mechanism) and completing the testing we are running on our knee with the University of Nebraska. Our next major milestone will be our FDA submission and from there an FDA approval.
Is there anything else that you would like to share regarding Monogram Orthopaedics?
We are commercializing highly complex technology and there is a bit of a learning curve to the industry and our technology. To accommodate people who are interested in learning more we regularly host webinars with live Q&A's as a management team.
To learn more visit Monogram Orthopedics or to invest visit the Monogram Orthopedics SeedInvest page.
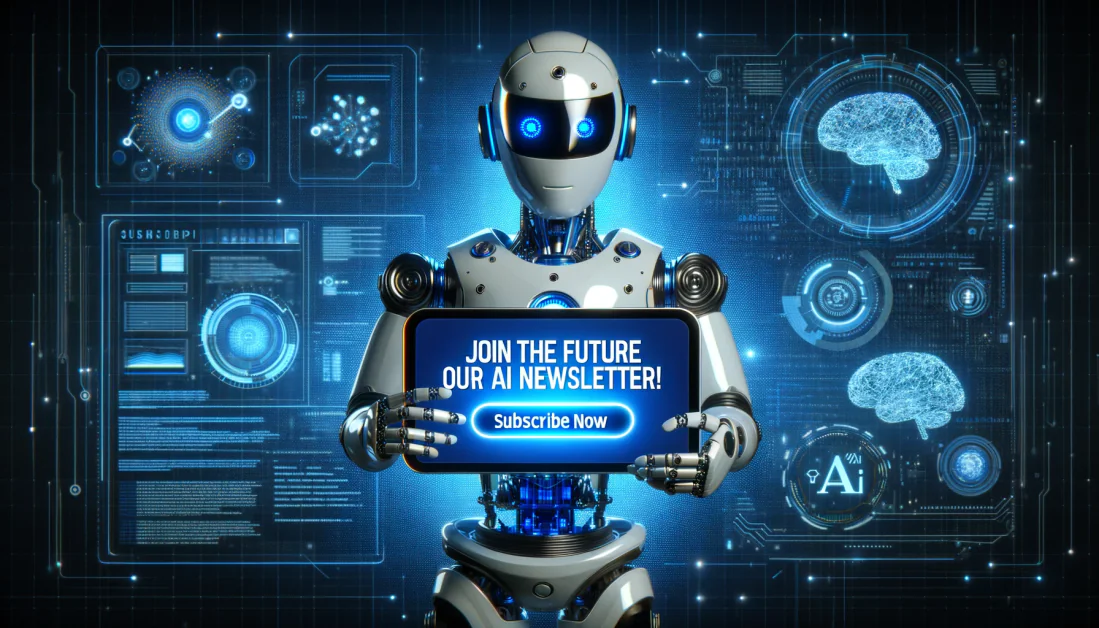
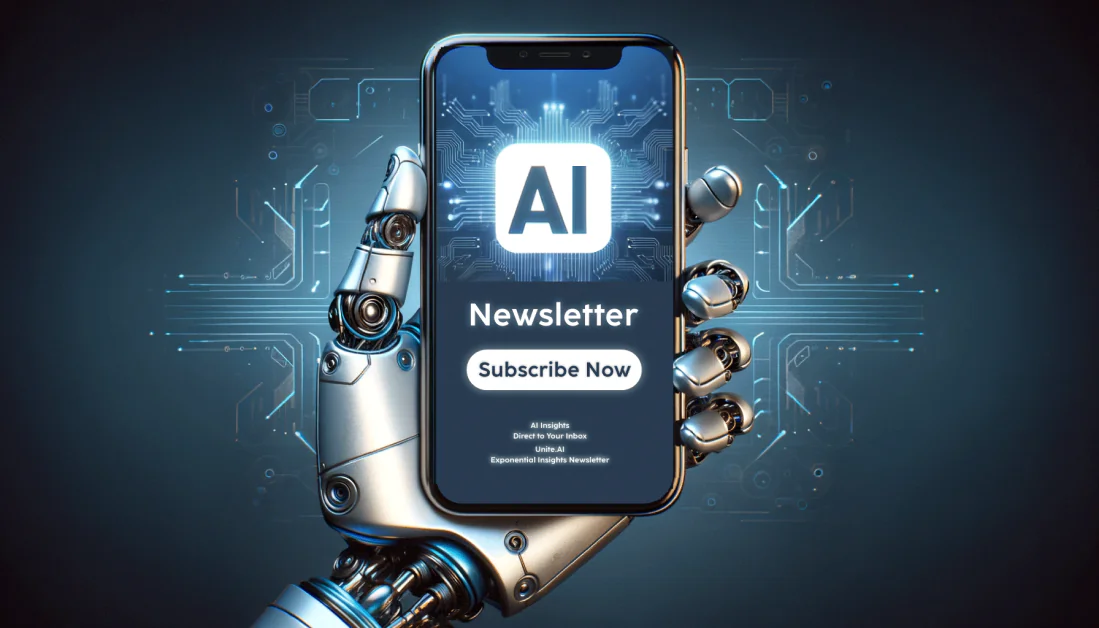