Interviews
Andrew Feldman, Co-founder & CEO of Cerebras Systems – Interview Series
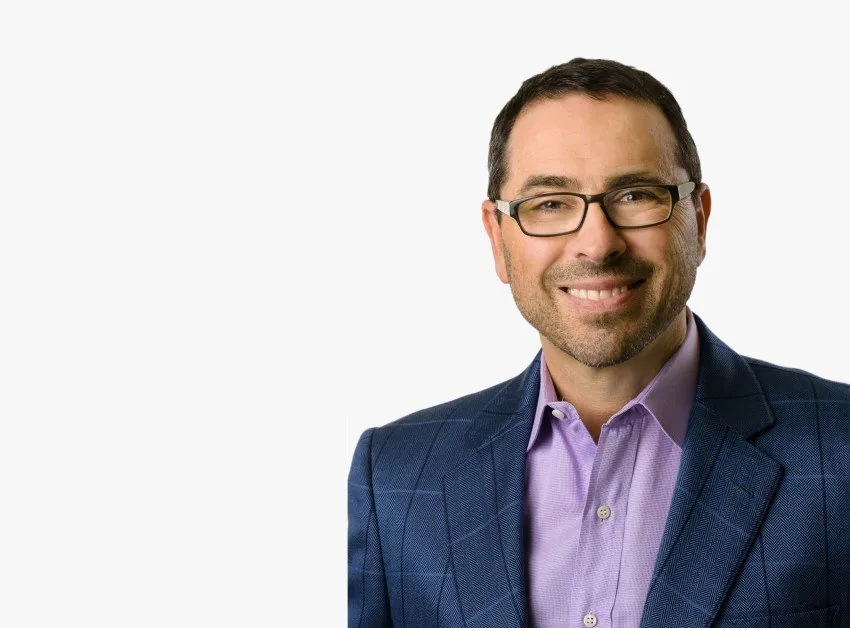
Andrew is co-founder and CEO of Cerebras Systems. He is an entrepreneur dedicated to pushing boundaries in the compute space. Prior to Cerebras, he co-founded and was CEO of SeaMicro, a pioneer of energy-efficient, high-bandwidth microservers. SeaMicro was acquired by AMD in 2012 for $357M. Before SeaMicro, Andrew was the Vice President of Product Management, Marketing and BD at Force10 Networks which was later sold to Dell Computing for $800M. Prior to Force10 Networks, Andrew was the Vice President of Marketing and Corporate Development at RiverStone Networks from the company’s inception through IPO in 2001. Andrew holds a BA and an MBA from Stanford University.
Cerebras Systems is building a new class of computer system, designed from first principles for the singular goal of accelerating AI and changing the future of AI work.
Could you share the genesis story behind Cerebras Systems?
My co-founders and I all worked together at a previous startup that my CTO Gary and I started back in 2007, called SeaMicro (which was sold to AMD in 2012 for $334 million). My co-founders are some of the leading computer architects and engineers in the industry – Gary Lauterbach, Sean Lie, JP Fricker and Michael James. When we got the band back together in 2015, we wrote two things on a whiteboard – that we wanted to work together, and that we wanted to build something that would transform the industry and be in the Computer History Museum, which is the equivalent to the Compute Hall of Fame. We were honored when the Computer History Museum recognized our achievements and added WSE-2 processor to its collection last year, citing how it has transformed the artificial intelligence landscape.
Cerebras Systems is a team of pioneering computer architects, computer scientists, deep learning researchers, and engineers of all types who love doing fearless engineering. Our mission when we came together was to build a new class of computer to accelerate deep learning, which has risen as one of the most important workloads of our time.
We realized that deep learning has unique, massive, and growing computational requirements. And it is not well-matched by legacy machines like graphics processing units (GPUs), which were fundamentally designed for other work. As a result, AI today is constrained not by applications or ideas, but by the availability of compute. Testing a single new hypothesis – training a new model – can take days, weeks, or even months and cost hundreds of thousands of dollars in compute time. That’s a major roadblock to innovation.
So the genesis of Cerebras was to build a new type of computer optimized exclusively for deep learning, starting from a clean sheet of paper. To meet the enormous computational demands of deep learning, we designed and manufactured the largest chip ever built – the Wafer-Scale Engine (WSE). In creating the world’s first wafer-scale processor, we overcame challenges across design, fabrication and packaging – all of which had been considered impossible for the entire 70-year history of computers. Every element of the WSE is designed to enable deep learning research at unprecedented speeds and scale, powering the industry’s fastest AI supercomputer, the Cerebras CS-2.
With every component optimized for AI work, the CS-2 delivers more compute performance at less space and less power than any other system. It does this while radically reducing programming complexity, wall-clock compute time, and time to solution. Depending on workload, from AI to HPC, CS-2 delivers hundreds or thousands of times more performance than legacy alternatives. The CS-2 provides the deep learning compute resources equivalent to hundreds of GPUs, while providing the ease of programming, management and deployment of a single device.
Over the past few months Cerebras seems to be all over the news, what can you tell us about the new Andromeda AI supercomputer?
We announced Andromeda in November of last year, and it is one of the largest and most powerful AI supercomputers ever built. Delivering more than 1 Exaflop of AI compute and 120 Petaflops of dense compute, Andromeda has 13.5 million cores across 16 CS-2 systems, and is the only AI supercomputer to ever demonstrate near-perfect linear scaling on large language model workloads. It is also dead simple to use.
By way of reminder, the largest supercomputer on Earth – Frontier – has 8.7 million cores. In raw core count, Andromeda is more than one and a half times as large. It does different work obviously, but this gives an idea of the scope: nearly 100 terabits of internal bandwidth, nearly 20,000 AMD Epyc cores feed it, and – unlike the giant supercomputers which take years to stand up – we stood Andromeda up in three days and immediately thereafter, it was delivering near perfect linear scaling of AI.
Argonne National Labs was our first customer to use Andromeda, and they applied it to a problem that was breaking their 2,000 GPU cluster called Polaris. The problem was running very large, GPT-3XL generative models, while putting the entire Covid genome in the sequence window, so that you could analyze each gene in the context of the entire genome of Covid. Andromeda ran a unique genetic workload with long sequence lengths (MSL of 10K) across 1, 2, 4, 8 and 16 nodes, with near-perfect linear scaling. Linear scaling is amongst the most sought-after characteristics of a big cluster. Andromeda delivered 15.87X throughput across 16 CS-2 systems, compared to a single CS-2, and a reduction in training time to match.
Could you tell us about the partnership with Jasper that was unveiled in late November and what it means for both companies?
Jasper’s a really interesting company. They are a leader in generative AI content for marketing, and their products are used by more than 100,000 customers around the world to write copy for marketing, ads, books, and more. It’s obviously a very exciting and fast growing space right now. Last year, we announced a partnership with them to accelerate adoption and improve the accuracy of generative AI across enterprise and consumer applications. Jasper is using our Andromeda supercomputer to train its profoundly computationally intensive models in a fraction of the time. This will extend the reach of generative AI models to the masses.
With the power of the Cerebras Andromeda supercomputer, Jasper can dramatically advance AI work, including training GPT networks to fit AI outputs to all levels of end-user complexity and granularity. This improves the contextual accuracy of generative models and will enable Jasper to personalize content across multiple classes of customers quickly and easily.
Our partnership allows Jasper to invent the future of generative AI, by doing things that are impractical or simply impossible with traditional infrastructure, and to accelerate the potential of generative AI, bringing its benefits to our rapidly growing customer base around the globe.
In a recent press release, the National Energy Technology Laboratory and Pittsburgh Supercomputing Center Pioneer announced the first ever Computational Fluid Dynamics Simulation on the Cerebras wafer-scale engine. Could you describe what specifically is a wafer-scale engine and how it works?
Our Wafer-Scale Engine (WSE) is the revolutionary AI processor for our deep learning computer system, the CS-2. Unlike legacy, general-purpose processors, the WSE was built from the ground up to accelerate deep learning: it has 850,000 AI-optimized cores for sparse tensor operations, massive high bandwidth on-chip memory, and interconnect orders of magnitude faster than a traditional cluster could possibly achieve. Altogether, it gives you the deep learning compute resources equivalent to a cluster of legacy machines all in a single device, easy to program as a single node – radically reducing programming complexity, wall-clock compute time, and time to solution.
Our second generation WSE-2, which powers our CS-2 system, can solve problems extremely fast. Fast enough to allow real-time, high-fidelity models of engineered systems of interest. It’s a rare example of successful “strong scaling”, which is the use of parallelism to reduce solve time with a fixed size problem.
And that’s what the National Energy Technology Laboratory and Pittsburgh Supercomputing Center are using it for. We just announced some really exciting results of a computational fluid dynamics (CFD) simulation, made up of about 200 million cells, at near real-time rates. This video shows the high-resolution simulation of Rayleigh-Bénard convection, which occurs when a fluid layer is heated from the bottom and cooled from the top. These thermally driven fluid flows are all round us – from windy days, to lake effect snowstorms, to magma currents in the earth’s core and plasma movement in the sun. As the narrator says, it’s not just the visual beauty of the simulation that’s important: it’s the speed at which we’re able to calculate it. For the first time, using our Wafer-Scale Engine, NETL is able to manipulate a grid of nearly 200 million cells in nearly real-time.
What type of data is being simulated?
The workload tested was thermally driven fluid flows, also known as natural convection, which is an application of computational fluid dynamics (CFD). Fluid flows occur naturally all around us — from windy days, to lake effect snowstorms, to tectonic plate motion. This simulation, made up of about 200 million cells, focuses on a phenomenon known as “Rayleigh-Bénard” convection, which occurs when a fluid is heated from the bottom and cooled from the top. In nature, this phenomenon can lead to severe weather events like downbursts, microbursts, and derechos. It’s also responsible for magma movement in the earth’s core and plasma movement in the sun.
Back in November 2022, NETL introduced a new field equation modeling API, powered by the CS-2 system, that was as much as 470 times faster than what was possible on NETL’s Joule Supercomputer . This means it could deliver speeds beyond what either clusters of any number of CPUs or GPUs can achieve. Using a simple Python API that enables wafer-scale processing for much of computational science, WFA delivers gains in performance and usability that could not be obtained on conventional computers and supercomputers – in fact , it outperformed OpenFOAM on NETL’s Joule 2.0 supercomputer by over two orders of magnitude in time to solution.
Because of the simplicity of the WFA API, the results were achieved in just a few weeks and continue the close collaboration between NETL, PSC and Cerebras Systems.
By transforming the speed of CFD (which has always been a slow, off-line task) on our WSE, we can open up a whole raft of new, real-time use cases for this, and many other core HPC applications. Our goal is that by enabling more compute power, our customers can perform more experiments and invent better science. NETL lab director Brian Anderson has told us that this will drastically accelerate and improve the design process for some really big projects that NETL is working on around mitigating climate change and enabling a secure energy future — projects like carbon sequestration and blue hydrogen production.
Cerebras is consistently outperforming the competition when it comes to releasing supercomputers, what are some of the challenges behind building state of the art supercomputers?
Ironically, one of the hardest challenges of big AI is not the AI. It’s the distributed compute.
To train today’s state-of-the-art neural networks, researchers often use hundreds to thousands of graphics processing units (GPUs). And it is not easy. Scaling large language model training across a cluster of GPUs requires distributing a workload across many small devices, dealing with device memory sizes and memory bandwidth constraints, and carefully managing communication and synchronization overheads.
We’ve taken a completely different approach to designing our supercomputers through the development of the Cerebras Wafer-Scale Cluster, and the Cerebras Weight Streaming execution mode. With these technologies, Cerebras addresses a new way to scale based on three key points:
The replacement of CPU and GPU processing by wafer-scale accelerators such as the Cerebras CS-2 system. This change reduces the number of compute units needed to achieve an acceptable compute speed.
To meet the challenge of model size, we employ a system architecture that disaggregates compute from model storage. A compute service based on a cluster of CS-2 systems (providing adequate compute bandwidth) is tightly coupled to a memory service (with large memory capacity) that provides subsets of the model to the compute cluster on demand. As usual, a data service serves up batches of training data to the compute service as needed.
An innovative model for the scheduling and coordination of training work across the CS-2 cluster that employs data parallelism, layer at a time training with sparse weights streamed in on demand, and retention of activations in the compute service.
There’s been fears of the end of Moore’s Law for close to a decade, how many more years can the industry squeeze in and what types of innovations are needed for this?
I think the question we’re all grappling with is whether Moore’s Law – as written by Moore – is dead. It isn’t taking two years to get more transistors. It’s now taking four or five years. And those transistors aren’t coming at the same price – they’re coming in at vastly higher prices. So the question becomes, are we still getting the same benefits of moving from seven to five to three nanometers? The benefits are smaller and they cost more, and so the solutions become more complicated than simply the chip.
Jack Dongarra, a leading computer architect, gave a talk recently and said, “We’ve gotten much better at making FLOPs and at making I/O.” That’s really true. Our ability to move data off-chip lags our ability to increase the performance on a chip by a great deal. At Cerebras, we were happy when he said that, because it validates our decision to make a bigger chip and move less stuff off-chip. It also provides some guidance on future ways to make systems with chips perform better. There’s work to be done, not just a wringing out more FLOPs but also in techniques to move them and to move the data from chip to chip — even from very big chip to very big chip.
Is there anything else that you would like to share about Cerebras Systems?
For better or worse, people often put Cerebras in this category of “the really big chip guys.” We've been able to provide compelling solutions for very, very large neural networks, thereby eliminating the need to do painful distributed computing. I believe that's enormously interesting and at the heart of why our customers love us. The interesting domain for 2023 will be how to do big compute to a higher level of accuracy, using fewer FLOPs.
Our work on sparsity provides an extremely interesting approach. We don’t do work that doesn't move us towards the goal line, and multiplying by zero is a bad idea. We’ll be releasing a really interesting paper on sparsity soon, and I think there's going to be more effort is looking at how we get to these efficient points, and how do we do so for less power. And not just for less power and training; how do we minimize the cost and power used in inference? I think sparsity helps on both fronts.
Thank you for these in-depth answers, readers who wish to learn more should visit Cerebras Systems.
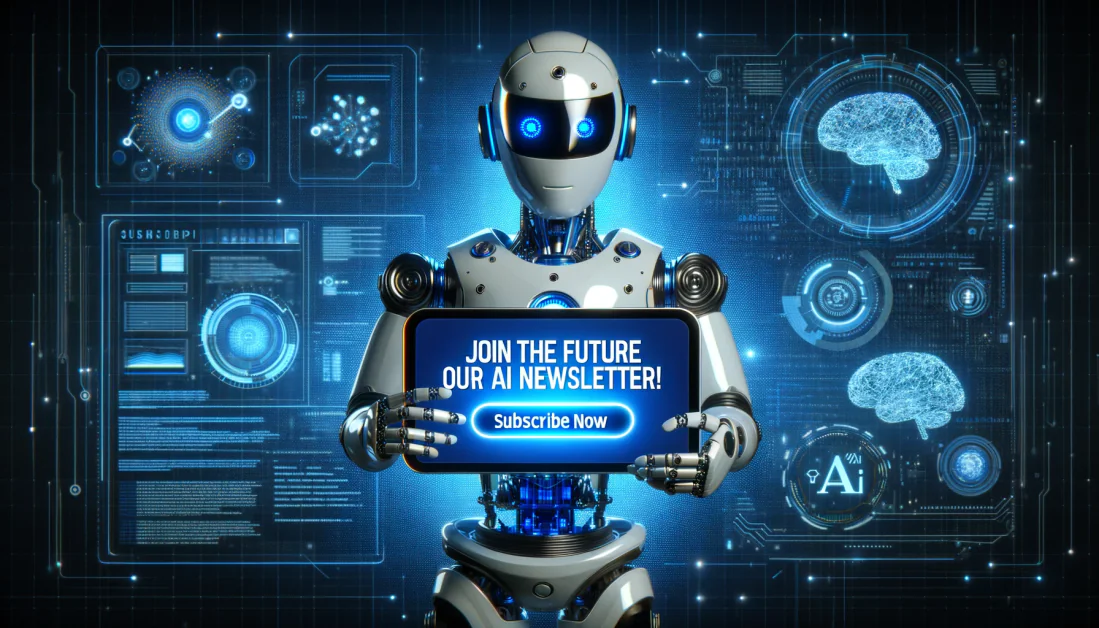
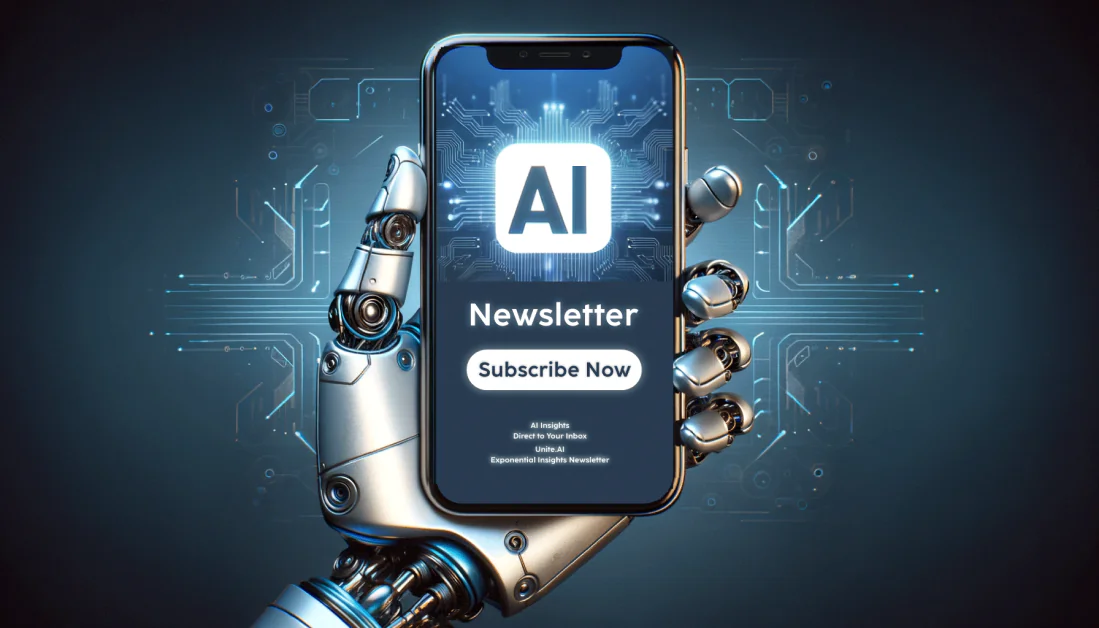