Artificial Intelligence
AI-Based Early Warning System Learns From Real Traffic Situations
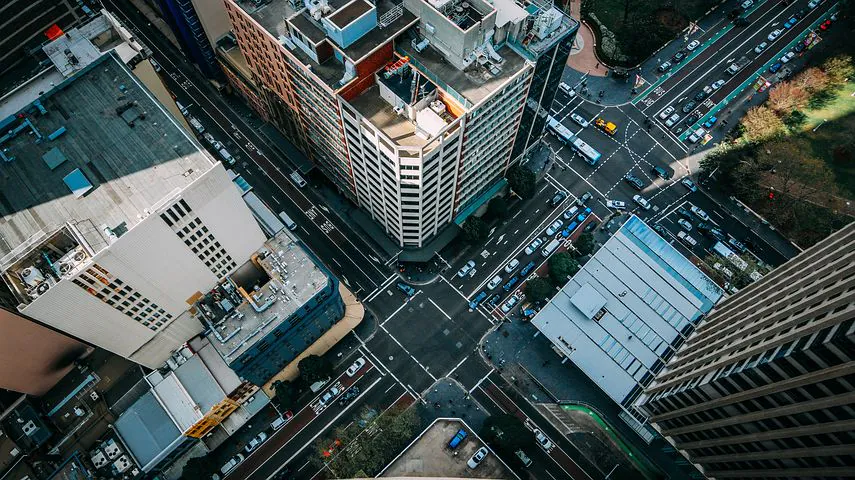
A new early warning system for vehicles developed by a team of researchers at the Technical University of Munich (TUM) utilizes AI to learn from thousands of real traffic situations. A study was carried out in collaboration with the BMW Group, demonstrating that the system would be beneficial to today’s self driving vehicles. If used, it can provide a seven-second warning against potential critical situations, which are situations that the cars cannot handle alone. The accuracy rate was shown to be 85%.
Current development efforts for self-driving vehicles use sophisticated models that enable the vehicles to analyze the behavior of all traffic participants. However, most of these models have still not demonstrated the ability to handle complex or unpredictable situations.
The team behind the new early warning system worked with Prof. Eckehard Steinbach, Chair of Media Technology and member of the Board of Directors of the Munich School of Robotics and Machine Intelligence at TUM.
The research titled “Introspective Failure Prediction for Autonomous Driving Using Late Fusion of State and Camera Information” was published in IEEE Transactions on Intelligent Transportation Systems.
The new approach uses AI to enable the system to learn from past situations. More specifically, these past situations were ones where self-driving cars reached their limit in real-world traffic. This takes place when human drivers must take control over the vehicle, either because the car signals for it or the human decides it is the safest option.
RNN and Seven-Second Warning
The new system relies on sensors and cameras that capture surrounding conditions, and status data like the steering wheel angle, road conditions, and weather are recorded. The AI system uses a recurrent neural network (RNN), which can process data to recognize patterns. Whenever the system detects a new driving situation that was not able to be handled previously, the driver is warned in advance.
“To make vehicles more autonomous, many existing methods study what the cars now understand about traffic and then try to improve the models used by them. The big advantage of our technology: we completely ignore what the car thinks. Instead we limit ourselves to the data based on what actually happens and look for patterns,” says Steinbach. “In this way, the AI discovers potentially critical situations that models may not be capable of recognizing, or have yet to discover. Our system therefore offers a safety function that knows when and where the cars have weaknesses.”
The technology was tested with the BMW Group, and autonomous vehicles on public roads were analyzed. This took place during 2500 situations where the human driver intervened, and the study demonstrated that the system is 85% accurate and can warn drivers up to seven seconds in advance.
The technology relies on large amounts of data since it is limited by how many situations the system has seen. The data was basically generated itself given the number of development vehicles currently on the road.
“Every time a potentially critical situation comes up on a test drive, we end up with a new training example.”
Because the data is centrally stored, the entire fleet can learn from it.