Cybersecurity
AI and Spam: How Artificial Intelligence Protects Your Inbox
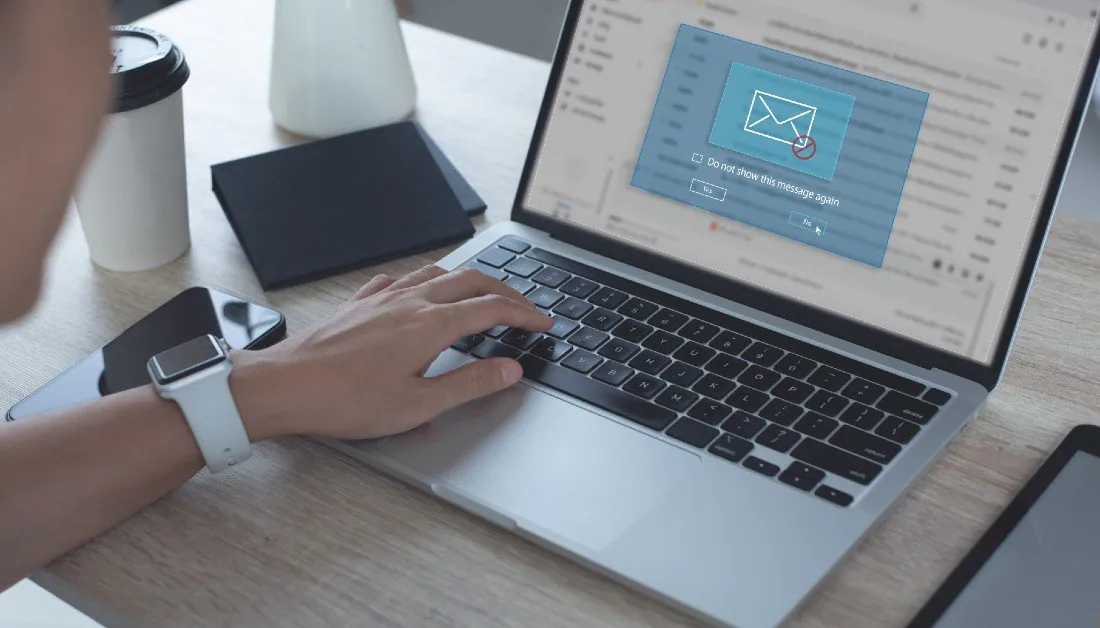
Conversations around AI often include its role in cybersecurity prevention. AI is a powerful, indispensable tool in fighting cyber threats, but it can also comb through email inboxes to eliminate spam. Many internet users see spam as an innocuous visual distraction, yet it can contain security risks, too. Implementing AI to fight incoming spam will reduce inbox numbers and keep users safe from malicious threats.
How Is AI Being Used to Fight Spam?
Industry leaders like Google are working on the macro level with their spam-filtering AI, TensorFlow. It aims to block spam — over 100 million messages daily — before individual malicious actors can breach targeted companies and individuals.
Spam is more than just an annoyance — it creates security and privacy risks. AI empowers other security measures, like firewalls and malware detection, to help prevent data breaches. Over time, however, defense lines like a firewall can deteriorate if email users ignore updating software. AI spam filtering can supplement business security measures as wear and tear open more gaps in a risk management plan.
Additional measures like AI spam filtering allow analysts and IT teams to execute maintenance. Data enters inboxes at an increasingly unprecedented rate. Spam sometimes outpaces relevant emails and it’s often too much for most humans to sift through or have time to handle. AI relieves humans of pressure in a digital climate operating at speeds beyond our cognition and wellness limits.
When AI filters spam, it relieves more technological burdens than pesky inbox clutter. For businesses, blocking or categorizing these messages saves networks storage space and money from manually designating incoming data.
How Does It Filter Spam Accurately?
Machine learning informs AI when it scans incoming emails. It looks for emails that signal red flags, such as:
- Malicious IP addresses and URLs
- Suspicious keywords
- Distrustful attachments or embedded content
- Inconsistent grammar, syntax and spelling, such as using symbols and numbers as letters
- Excessive use of special characters or emojis
With a database of countless references, it can examine email content for suspicious activity. Scanning can check links for fake login pages or verify signatures against employee databases. The more the AI analyzes, the more accurate it becomes in labeling emails as spam, automating once-manual processes like listing and blacklisting.
AI leverages several filtering algorithms to execute precise judgments on top of content and keyword assessment:
- Similarity-based: Filters compare incoming emails with pre-existing emails stored in servers.
- Sample-based: Templates of legitimate and non-legitimate spam emails allow AI to assess new emails.
- Adaptive: This algorithm reacts over time to adjust data categories. It compartmentalizes separate emails and compares potential spam against these more-specialized categories.
More complex algorithms will make AI more prepared during turbulent times. For example, spam content shifts based on global trends and international events. Spam emails contained false health information more during the pandemic as medical paranoia was at an all-time high. Events like these cause outliers in machine learning datasets, but they can be trained to consider these fluctuations.
What Evolutions Can We Expect?
Filtering comes at a risk — AI could accidentally misattribute secure emails as unsafe or vice versa. For example, harmful spam or phishing emails often strive to copycat or exploit credentials from reliable and familiar email structures and senders. Though some AI spam filters can notify recipients when it blocks a potential threat, eventually, AI will work more with human analysts to seek additional input.
Spam filtering will require rules to allow the AI to second-guess itself. Currently, AI systems might validate an email that looks like it comes from a secure source but is actually spam sent from a hacker’s highly trained algorithm. In time, AI spam filtering can become more attuned to nuances to eliminate false positives and identify when hackers employ social engineering in their spam distributions.
Refinement in natural language processing (NLP) could assess spam email content with improved finesse. AI relying on advanced NLP to filter out generic keywords and phrases will consider word vectors, also. Programming mathematical connections between words will allow AI systems to scan for intentions and connotations in written content, finding more links to potentially harmful representations from the internet’s historical data.
In addition to more competent AI filtering emails, it will supplement improved user training programs, especially in the workplace. Email users will understand how to categorize emails, especially as ambiguous, uncategorized graymail enters inboxes. Seminars and courses will evolve to involve human participants in training spam-filtering AI more directly.
AI’s Role in Organizing Email Inboxes
AI email filtering can manage incoming malware and protect email users from developing spam complacency. They appear as poorly written emails with unnatural links, but they jeopardize business and personal data.
Using AI to mitigate spam reduces breaches caused by human error and time spent on regular training when AI can cover most of the responsibility. With machine learning, AI will only increase its competence, saving inboxes from daily spam and unnecessary threats.
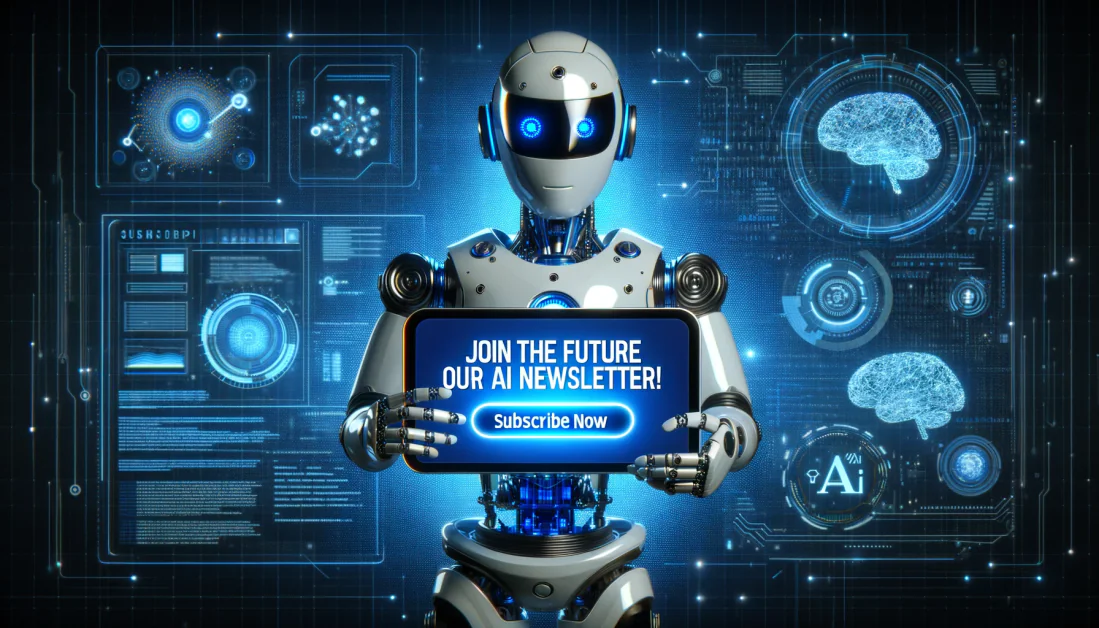
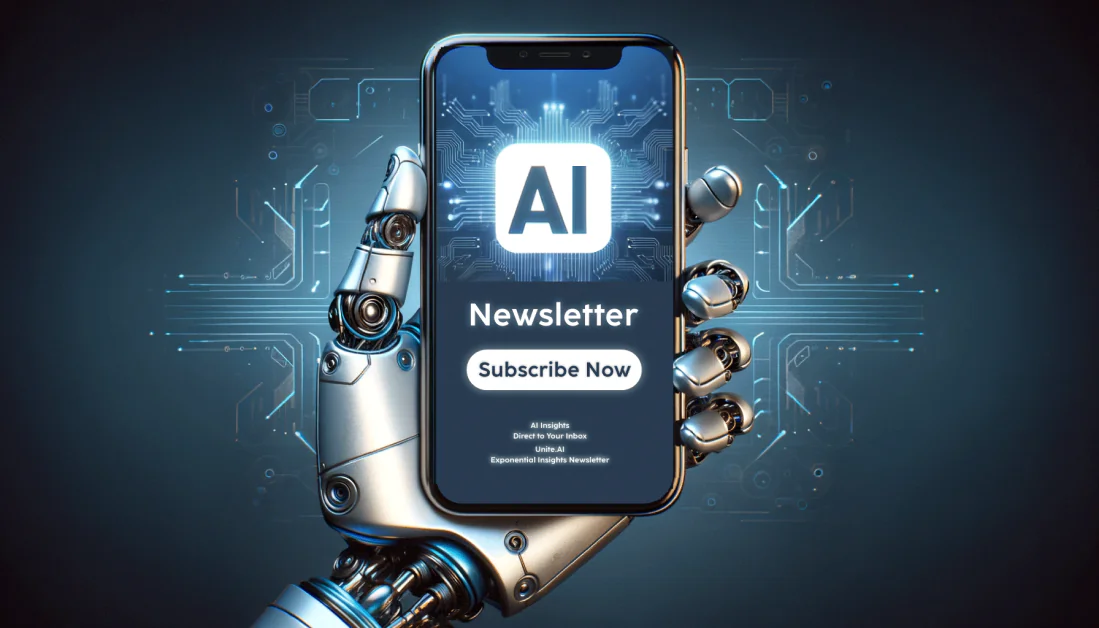