Healthcare
AI Algorithm Improves Accuracy and Costs of Medical Image Diagnostics
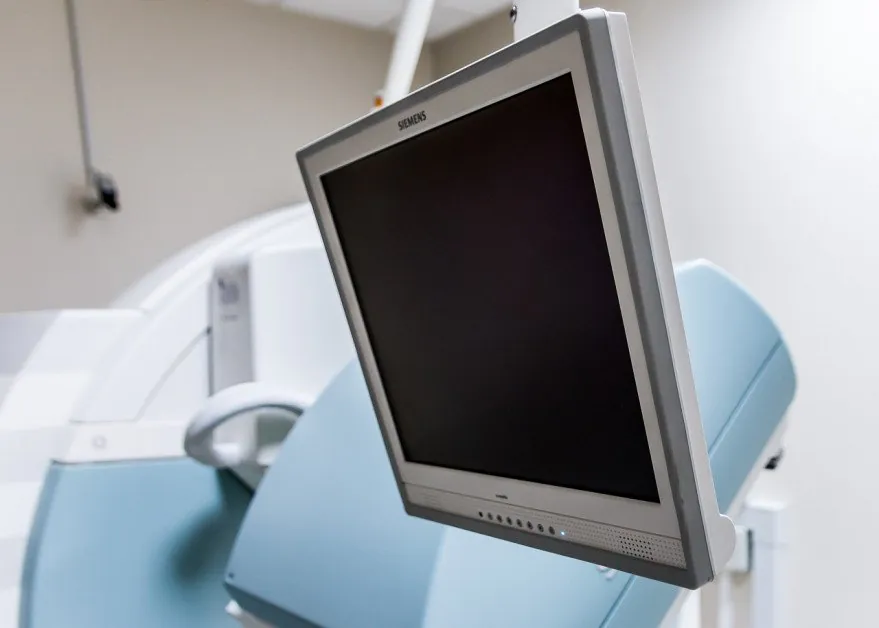
Medical imaging, which is a major part of modern healthcare, is one of the technologies that has been greatly improved through artificial intelligence (AI). With that said, medical image diagnosis relying on AI algorithms requires large amounts of annotations as supervision signals for model training.
Radiologists must prepare radiology reports for each of their patients to acquire these accurate labels for the algorithms. They then must rely on annotation staff to extract and confirm structured labels from the reports with human-defined rules and existing natural language processing (NLP) tools. This means the accuracy of extracted labels greatly depends on human work and the NLP tools, and the entire method is both labor intensive and time consuming.
REEFERS Approach
Now, a team of engineers at the University of Hong Kong (HKU) has developed a new approach called “REEFERS” (Reviewing Free-text Reports for Supervision). This new method can cut human costs by 90% by enabling the automatic acquisition of supervision signals from hundreds of thousands of radiology reports. This results in more accurate predictions.
The new research was published in Nature Machine Intelligence. It is titled “Generalized radiograph representation learning via ross-supervision between images and free-text radiology reports.”
The REEFERS approach brings us closer to achieving generalized medical AI.
Professor Yu Yizhou is leader of the engineering team at HKU’s Department of Computer Science.
“We believe abstract and complex logical reasoning sentences in radiology reports provide sufficient information for learning easily transferable visual features. With appropriate training, REFERS directly learns radiograph representations from free-text reports without the need to involve manpower in labeling.” Professor Yu said.
Training the System
To train REEFERS, the team uses a public database with 370,000 X-Ray images, as well as associated radiology reports. The researchers built a radiograph recognition model with just 100 radiographs and achieved 83% accuracy in predictions. The model was then able to achieve an 88.2% accuracy rate when the number was increased to 1,000. When 10,000 radiographs were used, the accuracy rose again to 90.1%.
REEFERS can achieve the goal by completing two report-related tasks. The first involves the translation of radiographs into text reports by first encoding radiographs into an intermediate representation. This is then used to predict text reports via a decoder network. To measure the similarity between predicted and real report texts, a cost function is defined.
The second task involves REEFERS first encoding both radiographs and free-text reports into the same semantic space. In this space, representations of each report and associated radiographs are aligned through contrastive learning.
Dr. Zhou Hong-Yu is first author of the paper.
“Compared to conventional methods that heavily rely on human annotations, REFERS has the ability to acquire supervision from each word in the radiology reports. We can substantially reduce the amount of data annotation by 90% and the cost to build medical artificial intelligence. It marks a significant step towards realizing generalized medical artificial intelligence, ” he said.