Artificial Intelligence
A ‘Detective’ AI Can Identify Obscure People From Multiple Sources
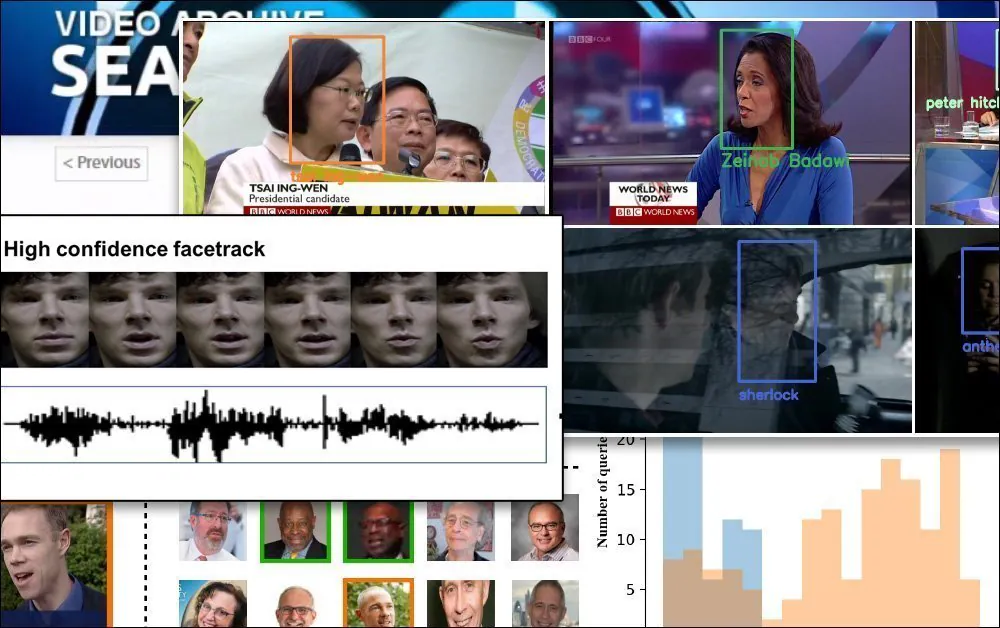
Researchers at Oxford University have developed an AI-enabled system that can comprehensively identify people in videos by conducting detective-like, multi-domain investigations as to who they might be, from context, and from a variety of publicly available secondary sources, including the matching of audio sources with visual material from the internet.
Though the research centers on the identification of public figures, such as people appearing in television programs and films, the principle of inferring identity from context is theoretically applicable to anyone whose face, voice, or name appears in online sources.
Indeed, the paper's own definition of fame is not limited to show business workers, with the researchers declaring ‘We denote people with many images of themselves online as famous‘.
Straight To Video
The researchers, from Oxford's Visual Geometry Group at Department of Engineering Science, outline the human-style investigative approach that has inspired the work:
‘Imagine that you are watching a video and encounter a new person. In order to confidently identify them, you would first look for clues of their name either in the video such as text on the screen, their name being mentioned in speech, or in a list of cast members from an internet archive. You might then find some evidence to verify that this name is correct, by searching for the person online.'
The methodology proposed by the paper is completely automated, and eliminates all additional manual labeling (discounting any that was performed by the providers of the online sources). The system was also proven to work well across three unrelated datasets without any need for domain adaptation.
Discussing the application of the work, the researchers note the exponential growth of unlabelled, opaque video data, and the need for new systems that can derive identity information from them without expensive human-led annotations:
‘[The] sheer scale of the data, coupled with a lack of relevant metadata, makes indexing, analysing and navigating this content an increasingly difficult task. Relying on additional, manual human annotation is no longer feasible, and without an effective way to navigate these videos, this bank of knowledge is largely inaccessible.'
An indexing engine of this nature opens up the possibility for search result hyperlinks that arrive directly at a point in the video where the search subject appears, as demonstrated in the proof-of-concept web search provided by the project.
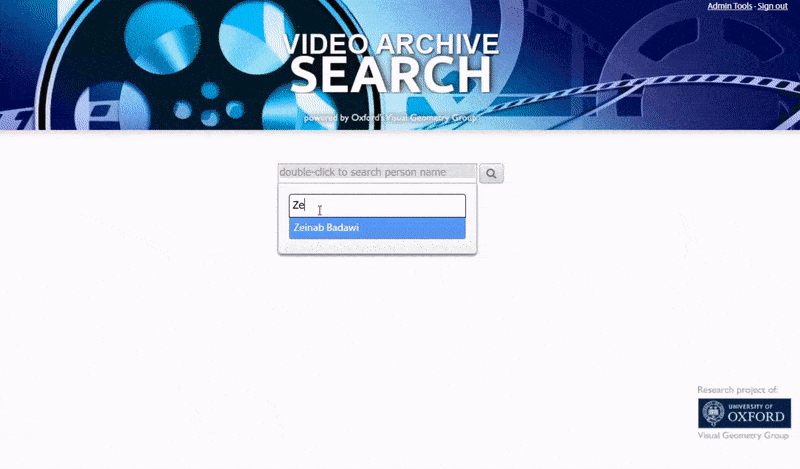
The Oxford system allows a search for instances of an identified person. The search result takes the viewer directly to the point in the video where the identified person appears, and the video can then be played from that point. Source: https://www.robots.ox.ac.uk/~vgg/research/person_id_in_video/
One of the ways that the system identifies ‘obscure' people is by the context of their association with others. Consequently, the search engine is well-tooled to search for multiple identities appearing in the same video:
Big And Little Fish
The system initially tackles the ‘low-hanging fruit' – people whose faces are so well-indexed in public-facing network resources that identifying them is relatively trivial, by matching metadata or OCR'd text in videos against public data resources such as IMDB lists. AI-interpreted text in video captions, credits and other forms of rasterized text in video is also leveraged to make the identification.
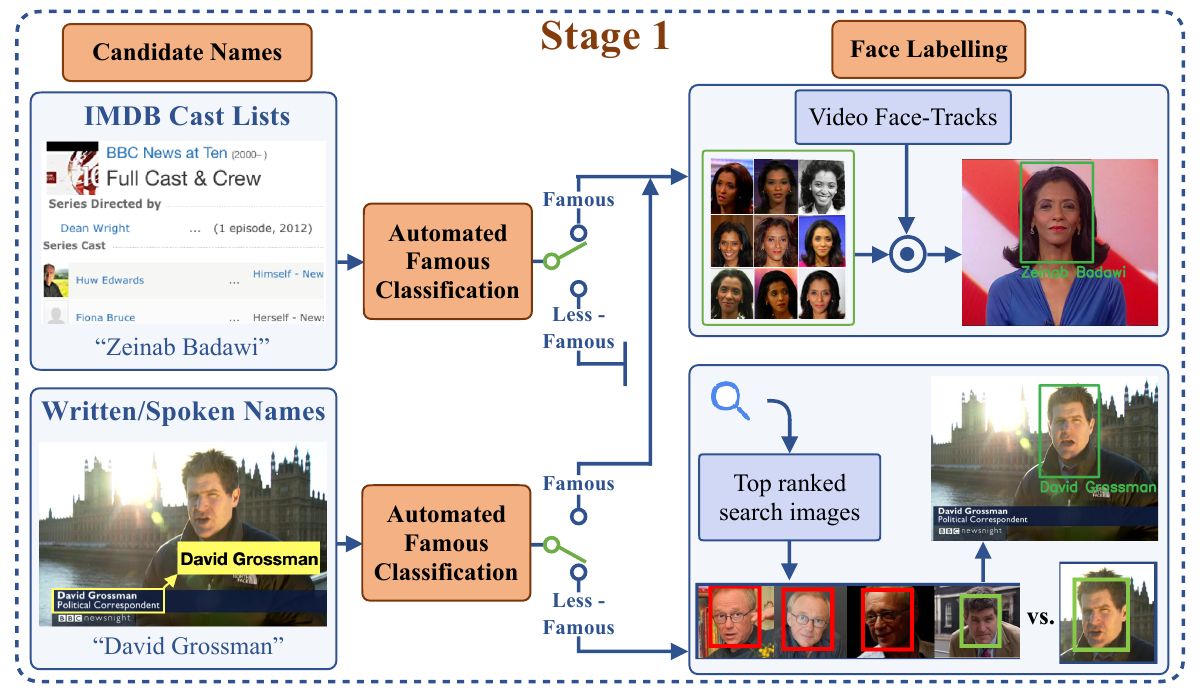
Candidate names for search can be auto-discovered by the system, based on optical character recognition (OCR) of rasterized text, or of actual text in other sources, such as cast listings. Thus, people can be indexed automatically without any prior queries run against their names by individual end users, and without prior participation in AI-enabled social networks. Source: https://www.robots.ox.ac.uk/~vgg/publications/2021/Brown21/brown21.pdf
Where overwhelming net-facing images and video confirm the identity of the person, the investigation affirms an identity. But where the person is more obscure, other methods are utilized, including audio taken from video tracks, which can be used as corroborating confirmation of an identity. Though not covered in the work, logically there is nothing to stop a framework of this nature also utilizing pure audio sources as well as audio components in video.
A Self-Propagating Identity Panopticon
In addition to generating candidate names from rasterized or pure text, speech recognition technologies are used in the Oxford project to recognize names that are merely spoken in audio content. Thus an identity can be initialized by one or two people merely mentioning a third person that is not present.
The safeguard that the Oxford project introduces is that the candidate must appear in the IMDB database, but removing this arbitrary stipulation broadens the potential scope of the system's capabilities considerably, since it relies entirely on web-scrapable resources.
Therefore, with a combination of sources including names derived from raster text, real text, speech-based mentions and very limited visual material, it becomes possible to identify individuals with a low visual network presence.
Technically, it also becomes possible to build up a profile of an individual to which no image or video footage has yet been associated, but to whom an image or video can eventually be attached when other factors correlate to a newly-ingested video source.
Test Datasets
The researchers used three datasets to evaluate the effectiveness of the system: MediaEval, which features Creative Commons social media-derived and community image resources (including Wikipedia and Flickr) captured between 2010-2015; the Oxford group's own 2017 Sherlock dataset, which features annotated video data from the popular modern-day BBC adaptation of the Conan Doyle classic character; and a new BBC videos dataset created specifically for the project, which uses various annotated news footage from the BBC.
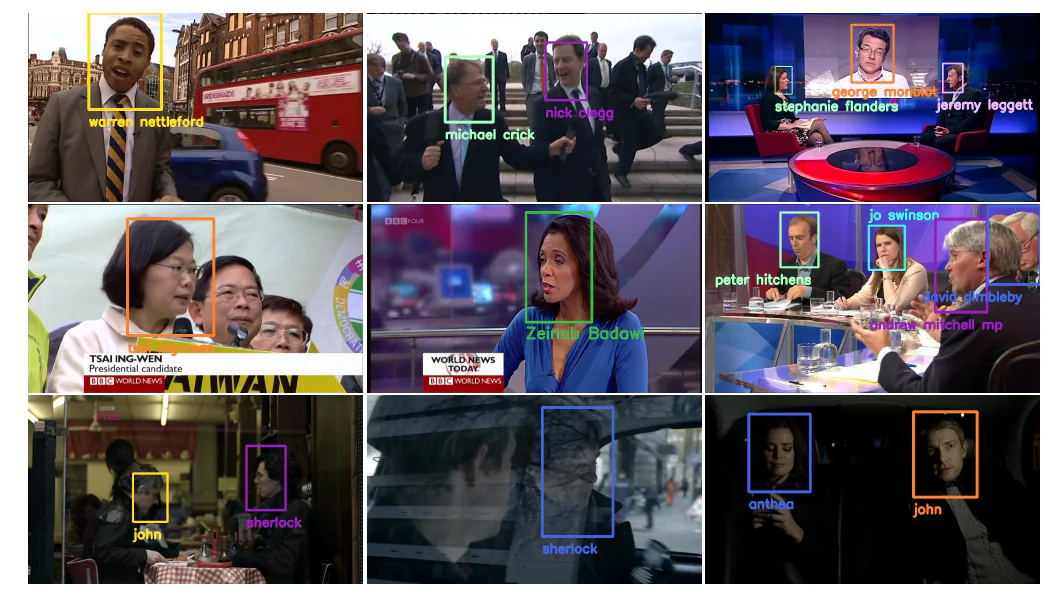
The system succeeds across a wide range of dataset environments, including occasions where the face is occluded by reflections or darkness.
The process also utilizes live image search rankings.
The results for the system produced high accuracy across the three models. In the case of the Sherlock dataset, the researchers were surprised to find that the new system improved 3-6% over a previous method that used support vector machines (SVMs) in a multi-way classifier, even though the nearest neighbor classifier used in the new work is a less powerful tool.
Implications
Most of the ethical or practical constraints in the Oxford project are self-imposed by the researchers, such as defining ‘fame' by the requirement that the identities discovered have a presence in the IMDB, and by testing the system solely against established academic datasets that respect Creative Commons licensing.
However, the essential architecture of the project depicts a generic method to not only identify ‘obscure' individuals that have low or zero visual presence on the internet (since a mere mention of a name can spawn an identity token that can be developed in time as necessary), but to actually create a matrix of individuals that is driven by nothing more than recursive and mechanistic curiosity, rather than by demand, or by the explicit presence of labeled data (such as social media photo uploads that contain PII metadata).
The project does not use geolocation data, or other forms of widely available metadata that might be found in contributing corroborative documents, such as geographic location information embedded by default in uploads to social media (where these are not stripped as a user preference). However, there is no apparent obstacle to using such extra dimensions of data to strengthen the corroborative process.
Whereas the Oxford project prunes outliers (identities that have almost zero presence, in addition to not being listed in the IMDB) in a way that's common in machine learning projects, such minimal information can arguably more effectively identify an unknown person than would happen if a higher amount of representative information about them was available. If outliers are exactly what you're looking for (i.e. individuals with little network footprint), sparse data can be highly indicative.
Availability
The Oxford researchers have encapsulated the project's functionality into a Google-like search engine that can be downloaded and installed on a local machine via Docker (though the installation instructions for the May 2021 paper currently contains out of date information for a Docker Tools requirement, which may hinder the process).
There is apparently no live online version that covers the implementation of the project across all three datasets, though results for the BBC news dataset can be freely interrogated at http://zeus.robots.ox.ac.uk/bbc_search/.