Regulation
Risks And Rewards For AI Fighting Climate Change
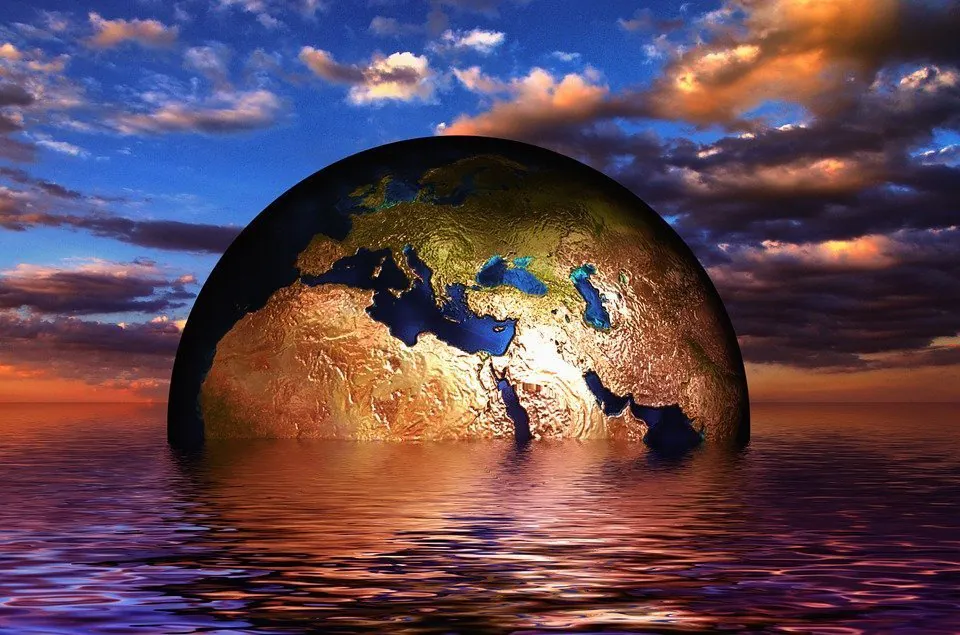
As artificial intelligence is being used to solve problems in healthcare, agriculture, weather prediction and more, scientists and engineers are investigating how AI could be used to fight climate change. AI algorithms could indeed be used to build better climate models and determine more efficient methods of reducing CO2 emissions, but AI itself often requires substantial computing power and therefore consumes a lot of energy. Is it possible to reduce the amount of energy consumed by AI and improve its effectiveness when it comes to fighting climate change?
Virginia Dignum, an ethical artificial intelligence professor at the UmeΓ₯ University in Sweden, was recently interviewed by Horizon Magazine. Dignum explained that AI can have a large environmental footprint that can go unexamined. Dignum points to Netflix and the algorithms used to recommend movies to Netflix users.Β In order for these algorithms to run and suggest movies to hundreds of thousands of users, Netflix needs to run large data centers. These data centers store and process the data used to train algorithms.
Dignum belongs to a group of experts advising the European Commission on how to make human-centric, ethical AI. Dignum explained to Horizon Magazine that the environmental impact of AI often goes unappreciated, but under the right circumstances data centres can be responsible for the release of large amounts of C02.
βItβs a use of energy that we donβt really think about,β explained Prof. Dignum to Horizon Magazine. βWe have data farms, especially in the northern countries of Europe and in Canada, which are huge. Some of those things use as much energy as a small city.β
Dingum noted that one study, done by the University of Massachusetts, found that creating aΒ sophisticated AI to interpret human language lead to the emissions of around 300,000 kilograms of the equivalent of C02. This is approximately five times the impact of the average car in the US. These emissions could potentially grow, as estimates done by a Swedish researcher, Anders Andrae, projects that by the year 2025 data centers could account for apporixmately 10% of all electricity usage. The growth of big data and the computational power needed to handle it has brought the environmental impact of AI to the attention of many scientists and environmentalists.
Despite these concerns, AI can play a role in helping us combat climate change and limit emissions. Scientists and engineers around the world are advocating for the use of AI in designing solutions to climate change. For example, Professor Felix Creutzig is affiliated with the Mercator Research Institute on Global Commons and Climate Change in Berlin and Crutzig hopes to use AI to improve the use of spaces in urban environments. More efficient space usage could help tackle issues like urban heat islands. Machine learning algorithms could be used to determine the optimal position for green spaces as well, or to determine airflow patterns when designing ventilation architecture to fight extreme heat. Urban green spaces can play the role of a carbon sink.
Currently, Creutzig is working with stacked architecture, a method that uses both mechanical modeling and machine learning, aiming to determine how buildings will respond to temperature and energy demands. Creutzig hopes that his work can lead to new building designs that use less energy while maintaining quality of life.
Beyond this, AI could help fight climate change in several ways. For one, AI could be leveraged to construct better electricity systems that could better integrate renewable resources. AI has already been used to monitor deforestation, and its continued use for this task can help preserve forests that act as carbon sinks. Machine learning algorithms could also be used to calculate an individual's carbon footprint and suggest ways to reduce it.
Tactics to reduce the amount of energy consumed by AI include deleting data that is no longer in use, reducing the need for massive data storage operations. Designing more efficient algorithms and methods of training is also important, including pursuing AI alternatives to machine learning which tends to be data hungry.