Artificial Intelligence
Human Image Synthesis From Reflected Radio Waves
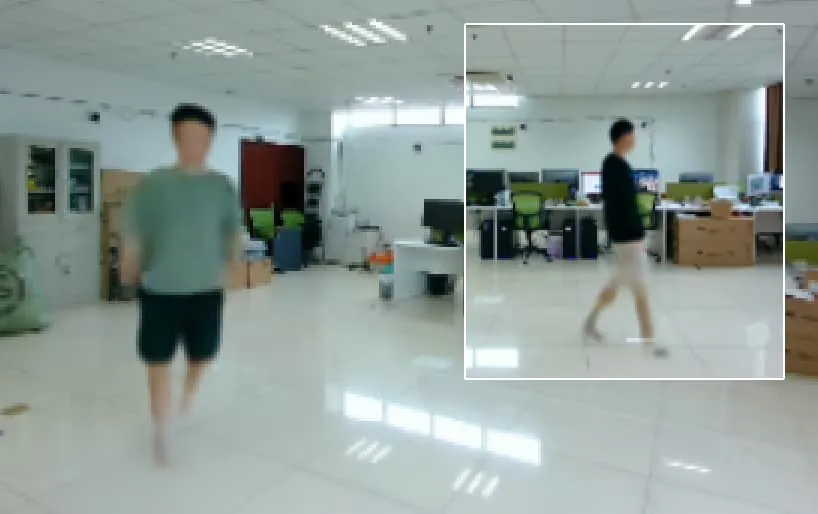
Researchers from China have developed a method to synthesize near photoreal images of people without cameras, by using radio waves and Generative Adversarial Networks (GANs). The system they have devised is trained on real images taken in good light, but is capable of capturing relatively authentic ‘snapshots' of humans even when conditions are dark – and even through major obstructions which would hide the people from conventional cameras.
The images rely on ‘heat maps' from two radio antennae, one capturing data from the ceiling down, and another recording radio wave perturbations from a ‘standing' position.
The resulting photos from the researchers' proof-of-concept experiments have a faceless, ‘J-Horror' aspect:
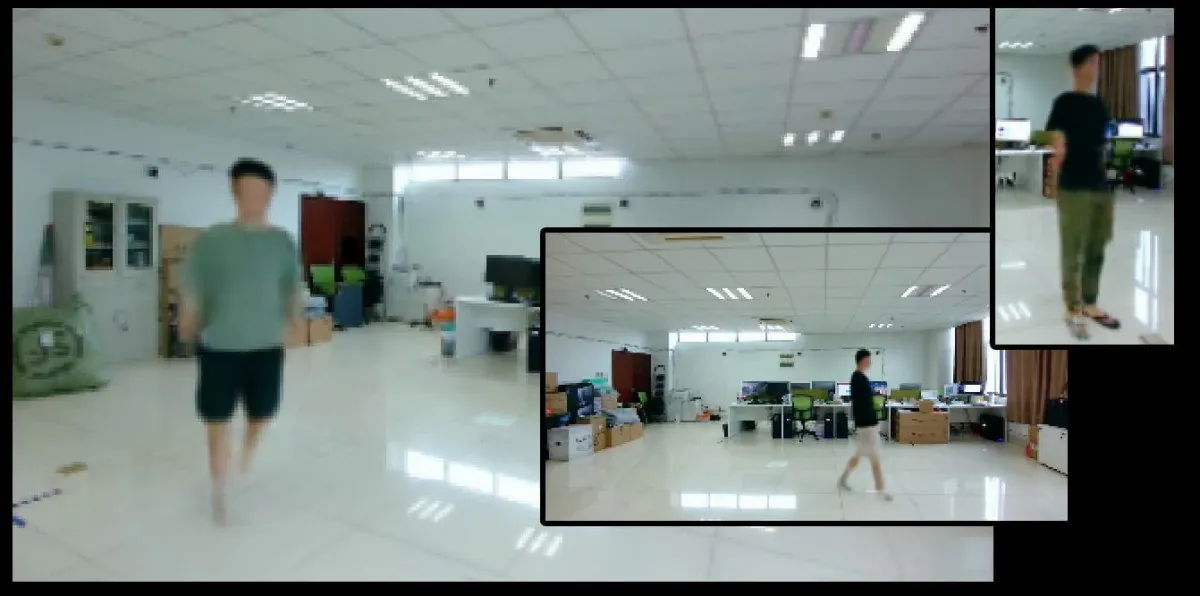
RFGAN is trained on images of real people in controlled environments and on radio wave heatmaps that record human activity. Having learned features from the data, RFGAN can then generate snapshots based on new RF data. The resulting image is an approximation, based on the limited resolution of the low frequency RF signals available. This process works even in darkened environments, and through a variety of potential obstacles. Source: https://arxiv.org/pdf/2112.03727.pdf
To train the GAN, dubbed RFGAN, the researchers used matched data from a standard RGB camera, and from the concatenated corresponding radio heatmaps that were produced at the exact moment of capture. Images of synthesized people in the new project tend to be blurred in a manner similar to early Daguerreotype photography, because the resolution of the radio waves used is very low, with a depth resolution of 7.5cm, and an angular resolution of about 1.3 degrees.
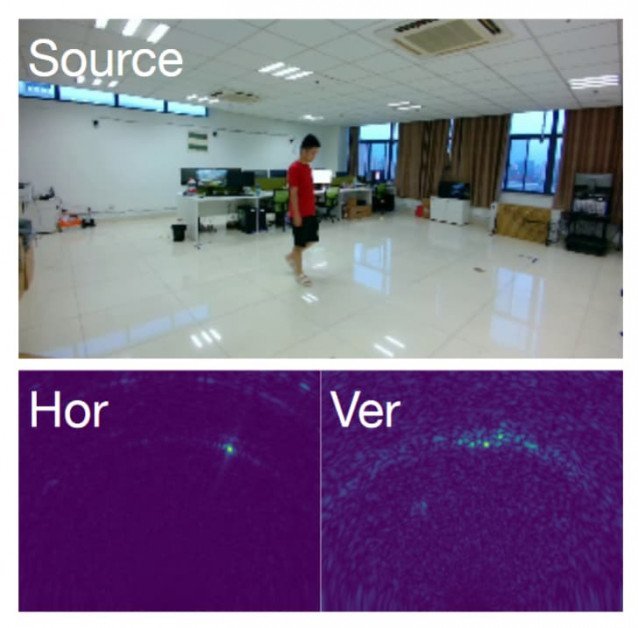
Above, the image fed to the GAN network – below, the two heatmaps, horizontal and vertical, which characterize the person in the room, and which are synthesized themselves inside the architecture into a 3D representation of the perturbed data.
The new paper, titled RFGAN: RF-Based Human Synthesis, comes from six researchers from the University of Electronic Science and Technology of China.
Data and Architecture
Due to the lack of any previous datasets or projects that shared this scope, and the fact that RF signals have not been used before in a GAN image synthesis framework, the researchers had to develop novel methodologies.
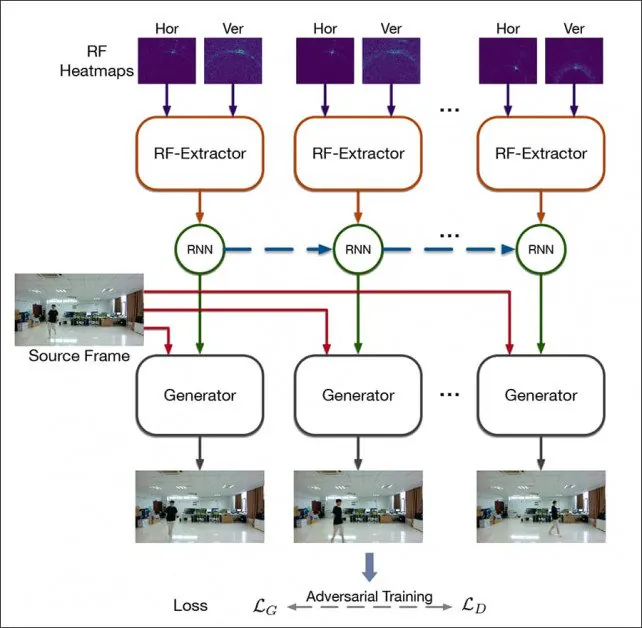
The core architecture of RFGAN.
Adaptive normalization was used to interpret the twin heatmap images during training, so that they correspond spatially with the captured image data.
The RF capture devices were millimeter wave (mmWave) radars configured as two antenna arrays, horizontal and vertical. Frequency Modulated Continuous Wave (FMCW) and linear antennae were used for transceiving.
The Generator receives a source frame as an input layer, with the RF fused (heatmap) representation orchestrating the network through normalization at the level of the convolutional layers.
Data
The data was collected from RF signal reflections from the mmWave antenna at a mere 20hz, with simultaneous human video captured at a very low 10fps. Nine indoor scenes were captured, using six volunteers, each of which wore different clothes for various sessions of the data-gathering.
The result was two distinct datasets, RF-Activity and RF-Walk, the former containing 68,860 images of people in varying positions (such as squat and walk), together with 137,760 corresponding heatmap frames; and the latter containing 67,860 human random walking frames, together with 135,720 pairs of associated heatmaps.
The data, according to convention, was split unevenly between training and testing, with 55,225 image frames and 110, 450 heatmap pairs used for training, and the rest held back for testing. RGB capture frames were resized to 320×180, and heatmaps resized to 201×160.
The model was then trained with Adam at a consistent learning rate of 0.0002 for both the generator and the discriminator, at an epoch of 80 and a (very sparse) batch size of 2. Training took place via PyTorch on a consumer-level sole GTX-1080 GPU, whose 8gb of VRAM would generally be considered quite modest for such a task (explaining the low batch size).
Though the researchers adapted some conventional metrics for testing the realism of the output (detailed in the paper), and conducted the customary ablation tests, there was no equivalent prior work against which to measure the performance of RFGAN.
Open Interest in Secret Signals
RFGAN is not the first project to attempt to use radio frequencies to build a volumetric picture of what's going on in a room. In 2019 researchers from MIT CSAIL developed an architecture called RF-Avatar, capable of reconstructing 3D humans based on radio frequency signals in the Wi-Fi range, under severe conditions of occlusion.
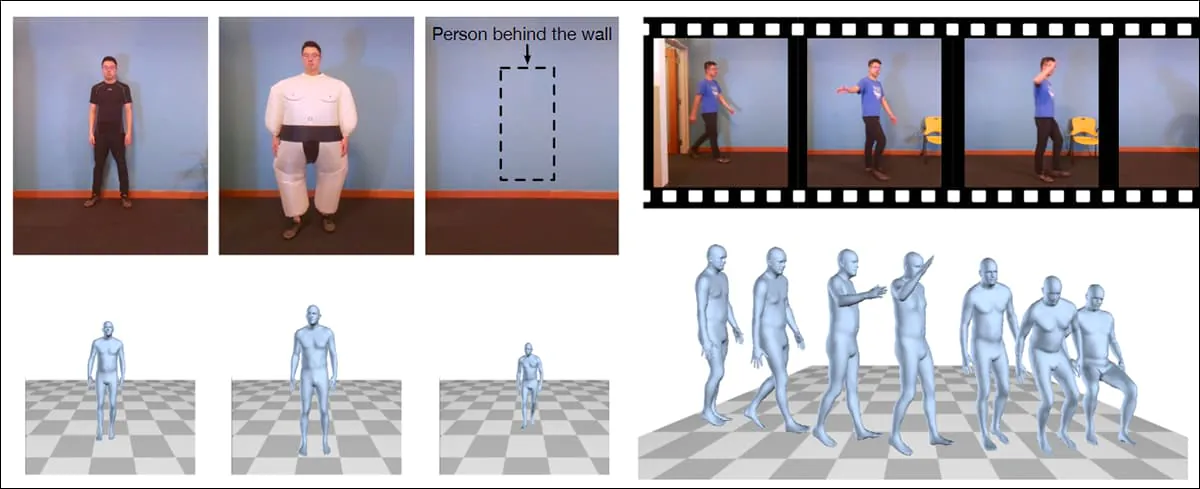
In the MIT CSAIL project from 2019, radio waves were used to remove occlusions, even including walls and clothes, in order to recreate captured subjects in a more traditional CGI-based workflow. Source: https://people.csail.mit.edu/mingmin/papers/rf-avatar.pdf
The researchers of the new paper also acknowledge loosely-related prior work around environment mapping with radio waves (none of it attempting to recreate photoreal humans), that sought to estimate human speed; see through walls with Wi-Fi; evaluate human poses; and even recognize human gestures, among various other goals.
Transferability and Wider Applicability
The researchers then set out to see if their discovery was over-fitted to the initial capture environment and training circumstances, though the paper offers few details on this phase of the experiment. They assert:
‘To deploy our model in a new scene, we do not need to retrain the whole model from the start. We can fine-tune the pre-trained RFGAN using very little data (about 40s data) to get similar results.'
And continue:
‘The loss functions and hyperparameters are the same with the training stage. From the quantitative results, we find that the pre-trained RFGAN model can generate desirable human activity frames in the new scene after fine-tuning with only a little data, which means our proposed model has the potential for being widely used.'
Based on the paper's details about this seminal application of a new technique, it's not clear whether the network that the researchers have created is ‘fit-trained' exclusively to the original subjects, or whether RF-heatmaps can deduce details such as color of clothing, as this does seem to straddle the two different types of frequencies involved in optical and radio capture methods.
Either way, RFGAN is a novel way of using the imitative and representative powers of Generative Adversarial Networks to create a new and intriguing form of surveillance – one that could potentially operate in the dark and through walls, in a way even more impressive than recent efforts to see round corners with reflected light.
8th December 2021 (day of first publication), 8:04pm GMT+2 – removed repeated word. – MA
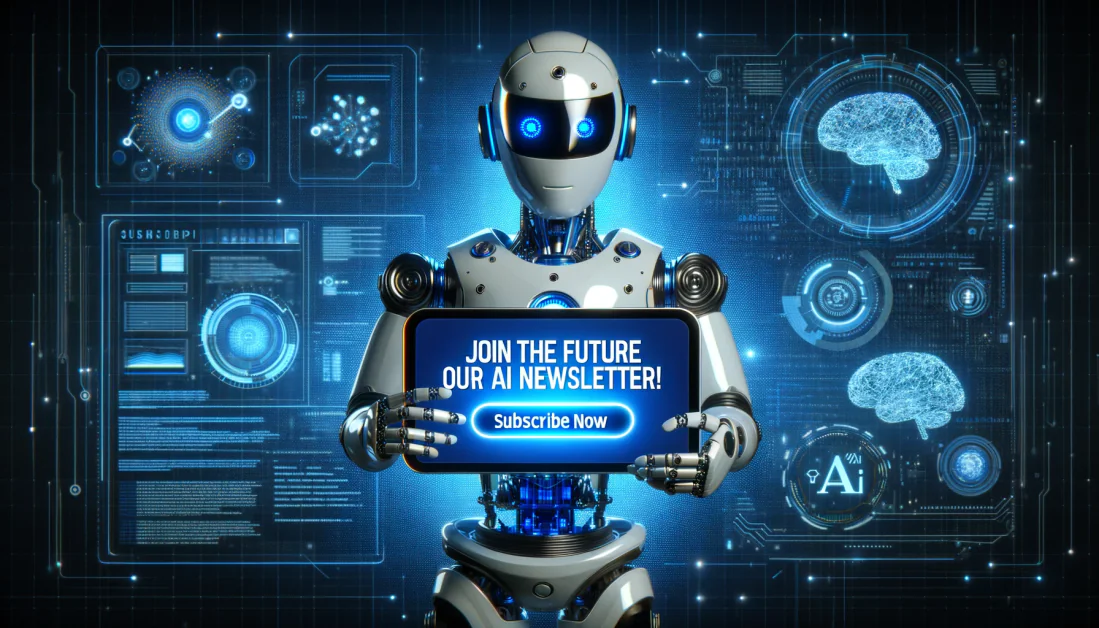
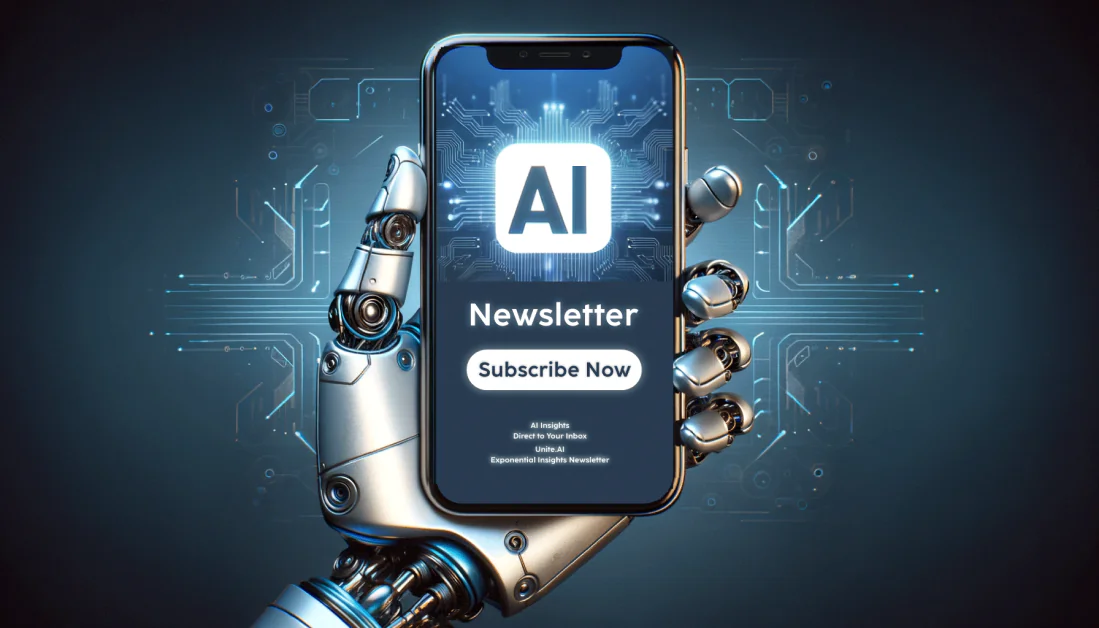