Interviews
Richard Boyd, Co-Founder & CEO of Tanjo Inc – Interview Series
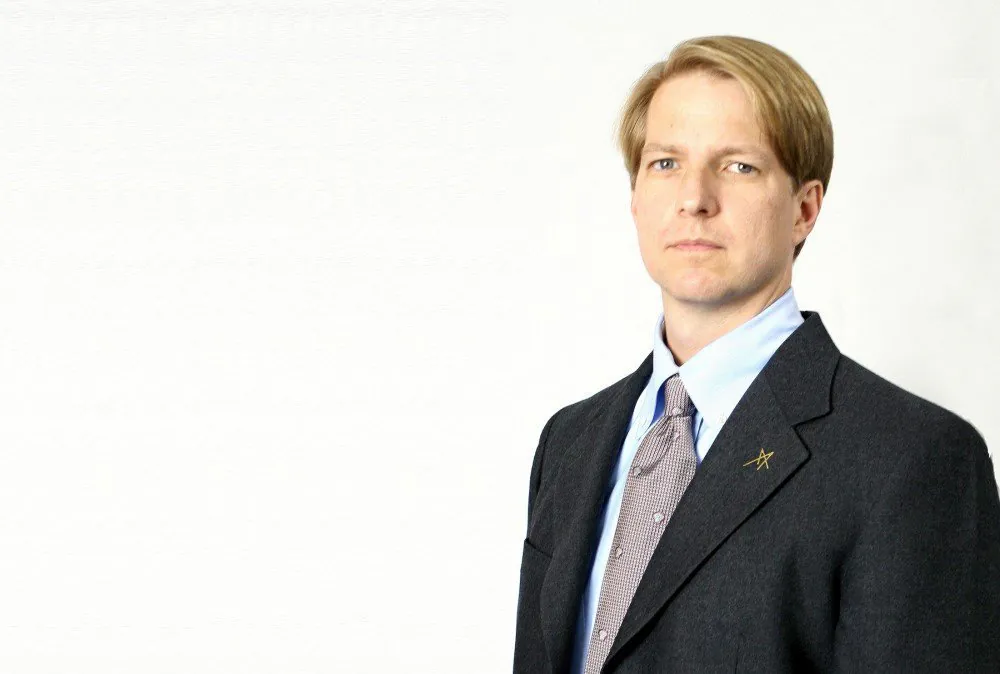
Richard Boyd is an entrepreneur, author and speaker on a range of topics from education to healthcare to virtual worlds, computer gaming, machine learning and human / computer interfaces. Over three decades, Richard has led or helped create some of the most innovative technology companies and services across several industries, including starting and serving as chief executive officer of four companies in North Carolinaâs Research Triangle Park region. Richard sold his last company to Lockheed Martin, and stayed on as Director of the Virtual World Labs.
Richard is co-founder and CEO of artificial intelligence and machine learning company Tanjo Inc based in North Carolinaâs Research Triangle Park region.
Youâve been working on VR since the 90s, and in 2001 you co-founded 3Dsolve. What were some of the initial projects that 3Dsolve worked on?
The most impactful first project for 3dsolve was helping the US Army Training and Doctrine Command (TRADOC) learn how to harness simulation learning for small unit tactical operations. We created the first “Level 4 interactive multimedia instruction” (IMI) course for the Army that passed TRADOC validation. Essentially this was harnessing the value of “safe practice in a fully simulated 3D environment for ground units” The first course was over 100 hours of instruction in a collaborative simulation 3D game-based world for the 25B10 MOS (military occupational specialty) for communications.
We were sending soldiers into Afghanistan and Iraq at the time and training them to work in DTOCs (digital tactical operations centers) when we had no DTOCS in the US to train them. 3Dsolve traveled to Fort Hood, Fort Gordon and various other facilities in order to find equipment, meet with subject matter experts and build the virtual DTOCs where soldiers could train. The validation results determined that soldiers using this method were trained in a shorter amount of time with a higher degree of knowledge (and class pass rates) than in any classroom methods used before. I think of this as the beginning of the serious games industry.
I also served on the ADL (Advanced Distributed Learning) Colab advisory board where the standards for reusable learning content were created. I worked with Phillip Dodds there on 3d standards for SCORM (Shareable content object reference model). Side note: Phillip was the guy who played the organ in the Spielberg movie Close Encounters of the Third Kind.
I also served on another international standards body called 3DIF, chaired by Intel and Boeing, where we created an international ECMA standard by the same name for 3d interchange formats. The idea was to finally capture all of the value in the 3D CAD models built for everything made in the World and translate it for use in serious games and 3D technical documents. It lives on in Adobe Acrobat and other platforms.
We continued to work with headsets and various VR peripherals, collaborating with industry pioneers Warren Robinett, Dr. Fred Brooks, Alan Kay and others. My co-founder David Smith created an entire open source platform together with Alan Kay called OpenCroquet, that still lives today.
How did life change for you after Lockheed Martin acquired 3Dsolve in 2007?
One of the other pioneering projects we worked on at 3Dsolve that caused Lockheed to buy us was a simulation of an entire Los Angeles class submarine. At the time the navy was still setting entire ships aside for training. We pioneered the idea of “Total Ship Simulation”, replicating the entire submarine in a multiplayer game environment. We used the Epic Games Unreal engine and really transformed the training for these vessels. At Lockheed we created sims of destroyers, the Littoral Combat Ship as well as all of the sub systems.
It was a challenge at first adapting from a game technology company to the extra layers of oversight and reporting necessary in a 100 year old defense contractor. We learned how to create our own reality. I formed an informal organization called Virtual World Labs and modeled it after the famous LM Skunkworks in California. In fact, the Skunkworks became a member of VWL . We learned in the first year that any time you come up with a patent proposal you get a check, and another one when it is granted. So, this became our incentive program. We sat around inventing stuff in AR, VR and AI. By the end of my 5-and-a-half-year stint there we had accumulated over 100 patent applications in a small group of about 40 people.
One of the most fun and relevant programs was the creation of the DOD Virtual World Framework. We had been part of several large-scale joint war game exercises and watched the frustrations over lack of interoperability among proprietary systems that needed to work together in these big live, constructive and virtual simulation exercises. Our first reaction was that this was a solved problem…It's called the Internet! If the acquisition community could just get everyone to adhere to web services we can build better integrated training systems. And WebGL had just passed muster with the World Wide Web Consortium. The time had come for a shakeup of business as usual. The Pentagon put out a request for proposal seeking a “common virtual world framework” for integrated simulation. The head of that program at the Pentagon was a creative former air force pilot named Frank Digiovanni. We called him D9. He reminds me a lot of the stories of Col John Boyd who pushed for creative destruction of our fighter jet programs and thinking in the air force.
The problem was, D9 explicitly told his acquisition team that he “didn't want any Lockmart like companies” to build that new framework. But David and I went in there along with the usual list of about 17 vendors bidding on the program and we won it. We learned afterwards that everyone else was showing up, predictably, with some proprietary solution and trying to get the government to adopt it. We showed up for our verbal presentation at the Reagan building in DC with nothing, but said that the answer to this deep problem lies in the architecture of the Internet. We said we could design it in a few months and have a working prototype in six. We also said it should be open source. We won “hands down slam dunk” according to D9, because our approach was so fresh and different and “outside the box”.
When I returned to Orlando to explain this new win to the leadership of our Lockheed Martin division I was kind of called out on the carpet. I was congratulated, but then they asked about the open source part. “What will this mean to our existing constructive sim business?” they asked. My reply -“Oh, it will completely disrupt it.” There was a pause, then the inevitable question “So, how will we make money? What is the business model?” My reply – “The business models will be legion.” I still savor the confused frowns that reply evoked. I went through all of the ways Red Hat managed to build a multibillion-dollar business on the back of free software, but I don't think they ever got comfortable with the disruption.
My title at the time was Director of Emerging and Disruptive Technology, along with my title of Chief Architect of Virtual World Labs. I worked for the next year trying to get Lockheed to embrace more self-disruption and Schumpeter creative destruction. I described innovation in big organizations like Lockheed as being similar to childbirth. People love the idea of having children. It is good for society and very rewarding. Children are our future. But through the wrong lens, children can also be considered parasites. Beginning when they first take purchase in the womb, they begin taking resources away. If it were not for the sheltering conditions of the womb, the Mother's antibodies would come out to destroy the baby. Innovation in Lockheed was like that. Everyone wants and talks about innovation, but no one wants to sacrifice their resources for it when the payoff is so far away. (See my white board animation about how innovation is like childbirth.)
During your time at Lockheed Martin one of the patents you co-authored sounds like something out of a science fiction blockbuster, called the holodeck. What exactly is the holodeck?
In 2009, I was invited out to Los Angeles by James Cameron during production of his movie Avatar. We had worked with Jim before (on “The Abyss”) and he wanted to show me his new 3d camera he had invented with Vince Pace (who we also knew from The Abyss). But the thing that really captivated me was the virtual set inside the huge Hughes aircraft hangar. I spent a lot of time in there with a little flat panel screen just wandering around the virtual world of Pandora. I wrote about this for Armed Forces Journal and conceived with David Smith the idea of building a big virtual training battle ground the size of a football field. at the time we were working on a program called Future Immersive Training Environment (FITE) for the Marines. In that program the marine would wear a laptop on their back and the headmount. All of this extra gear really caused some concern about negative training. I will never forget the first sergeant who strapped it on and said, “we have to train like we fight right?” and then dove on the ground and rolled, smashing all of the electronics to chunky useless bits. The Holodeck concept was more like James Cameron's Volume for filming; where the actors have light tracking suits and all of the instrumentation is around them. The head mounted display was still necessary but was wireless and lightweight. More like today's Oculus Quest. We even figured out a way to do it outside in sunlight.
In 2015 you wrote that we should not worry so much about machines taking over, but instead we should figure out how to achieve the right balance between humans and automation to optimize outcomes. Do you still feel society is overly concerned about AGI or machines taking over?
I think when really smart people with an abundance of expertise in this space (like Ray Kurzweil, Stephen Hawking, Elon Musk, James Cameron and Bill Gates) express concerns, we should all pay attention and track the progress towards artificial general intelligence and the implications for society. If we have learned anything recently it is that domain expertise matters, and we should always heed warnings from people with deeper expertise than our own.
Having said that, for the foreseeable future we are seeing more incremental disruption that merits immediate attention and action. My distilled quote about the 21st century imperative of achieving the right balance between humans and automation to optimize outcomes is a critical issue right now. I really think anyone who does not get that right is doomed to be irrelevant soon, not just not competitive. When JP Morgan replaced 320,000 hours a year of legal review of loan agreements with a machine learning system called COIN, they disrupted themselves and immediately created a $300 million benefit to their bottom line. And that benefit is now an annuity. Any of their competitors who still have that cost cannot hope to compete.
I believe this is true and imperative for companies, governments, even individuals. I am on the board of a community college in North Carolina with 70,000 students. I am constantly trying to guide students and our curriculum towards those jobs that will still be performed by humans five years from now. When I find students, who want to go into radiology I explain to them that machines are already better than humans at reading xrays. Consider a new field or how that field is likely to change with that reality. This isn't futurism. It's Nowism.
Youâve stated that humans think linearly and that machines think exponentially. Clearly you are an exponential thinker, why is it so difficult for humans to think exponentially?
70% of Americans cannot read and comprehend the science section of the New York Times (Michigan State study). Authors like Dan Ariely, in his best seller Predictably Irrational, and others talk about how we humans just are not good at statistical thinking. Exponential and logarithmic thinking are also not very universal. My mentor and hero Alan Kay has a great Ted Talk on universals and non-universals in education. I wrote about this in an article In the Getting Smart website about Rethinking Education from First Principles. Essentially abstract and deductive reasoning are difficult unless taught. We absolutely have an education issue that is hampering our ability to grasp the progress of Moore's Law or the likely spread of a pandemic.
The current pandemic shines another bright light on the implications of having leadership who cannot think exponentially (or heed expertise).
Youâve worked in VR since the 90s, how do you feel about some of the current VR consumer applications such as the Oculus Quest?
Every time I see the roller coaster of VR hype heading back up the slope of the track I start reminding everyone of the three main constraints that are preventing widespread adoption.
- Some humans will forever be physically unable to enjoy stereoscopic 3D VR.
- The friction of setup and connection make it an experience that not many will find delightful.
- The brittleness of the systems mean that only expert hobbyists will want to tinker with it and trouble shoot failed connections
Humans haven't had an upgrade in a long time (since the Pleistocene by my reckoning) and some of us have a very difficult time adapting to stereoscopic 3d displays. A significant portion of the population is never going to get comfortable with immersive VR because of how they are physically wired. So, setting them aside for a moment, we are left with the second big issue:Â the horrible friction of tethering to these devices. Way too much cabling and tuning before one can get into an experience. And third is the fragility and brittleness of all of these extra dongles and connectors.
The Oculus Quest vastly exceeded my expectations by completely blowing out the second and third constraints. In my family we spend time in Oculus Quest content almost every day. This is, in my view, the big breakthrough that VR needed. Now we just need to go the last mile and see how we can adapt the tech to meet those who have physical constraints that prevent enjoyment of VR.
What was your inspiration behind launching Tanjo?
I discovered machine learning in 2009 while I was running Virtual World Labs at Lockheed Martin. Machine learning already existed, of course, but that was the year I fully understood how far it had come, and how it was fundamentally different from the âArtificial intelligenceâ we had been using in computer games and DOD simulations before then.
I now think of AI as advancing in 3 stages. In the first stage, that lasted from around 1958 until 2009 (my arbitrary marker) we wouldnât ask a computer to compute something until humans understood it completely and could break it down into brittle little logic gates and if/then statements. We would then feed that into the computers as finite state machines or hierarchical behavior trees and run the programs. In the end, it was all just code. Nothing mystical about it.
The next phase is machine learning, where a human doesnât necessarily even understand how to tell a machine to drive a car. Now we just feed a massive set of training data to a group of well-designed machine learning libraries that then infer their own understanding. Today a machine learning system can just watch 100 hours of video and go out and drive an autonomous vehicle flawlessly anywhere. (I usually make the joke âanywhere but in Romeâ)
At Tanjo we are using machine learning in short burst projects to give banks and institutions of higher education and fortune 2000 companies the intelligence amplification and automation that is transforming how they work. We routinely see returns on investment of 10x from our implementations. And that return is usually an annuity. How many technology investments have we seen before this that create those kinds of productivity gains? We have had validated ROI measurements of as much as 600x; and one embarrassing result of 1600x. We donât even use the last one as a case study because it feels too hyperbolic.
Could you discuss the Tanjo Animated Personas (TAP), and how it works?
Our big breakthrough came when we realized that these amazing, weird machine intelligence systems we were building looked at people the same way that they looked at information objects. We ran an early experiment with a set of training data from a popular dating app. Our little mini machine learning brain created interest graphs and sentiment maps of each person from their data exhaust that emerged looking something like a Myers Briggs profile. We thought briefly about making a machine learning dating app in 2014. It was a very brief consideration, because it didnât meet the lofty goals, we had for doing meaningful work.
Instead, we called it the âEmpathy Engineâ and built what we called âTanjo Animated Personasâ from these machine learning patterns of human behavior.
The analyst firm Gartner gave us a “Cool Vendor Award” in 2018 for this breakthrough. We are helping market researchersâ model and understand (and hopefully forge deeper meaningful conversations with their customers; as well as using it to model populations of people to study health and wellness. For example: we can create a synthetic population model of a zip code or a county and simulate which interventions and messages encourage better behavior to reduce the spread of a virus, or to lower obesity, smoking etc.
Do you use supervised learning to educate the Tanjo?
The balance struck between humans and machines is as important in the input as it is in the output of these systems. Human supervision absolutely helps with training the “Brain” of one of our Machine learning systems faster. When we created the NC brain that will tie all 58 community college in North Carolina together we worked with faculty and administrators at some of the top colleges here to ensure that its ranking of different areas of knowledge and how it sorted the content was valid.
One of the Tanjo products is the ContractBot for contract analysis. What is the ContractBot and what types of enterprises is it primarily designed for?
We created Contractbot initially for the accounting industry. In 2017 FASBI (Financial Accounting Standards Board Interpretations) was releasing new rules around revenue recognition and lease recognition for business. Accounting firms were holding conferences around the country trying to prepare themselves and their clients for these changes. With our machine learning lens, we realized this was a perfect opportunity for a narrowly focused machine learning system to work alongside accountants to dramatically increase speed of analysis as well as increase accuracy. We trained a system on over four million contracts: everything from a one page scanned in, hand-scrawled purchase order, to contracts with a hundred pages of warranties and disclaimers and milestone payment descriptions. It learned very quickly to understand the language and sort the documents or sections of documents and apply the business rules to almost instantly performa analysis that would take a human accountant all day.
This project and others are case studies we provide to encourage anyone in business today to take the new machine/human balance lens and take a close look at every activity to determine what is the right mix of human and machine effort to optimize their business.
When JP Morgan used this approach to eliminate 320,000 hours annually of loan analysis, they not only realized a 100x return on their investment that year, but will receive that annuity payout every year going forward. Any competitor of theirs who is still doing “business as usual”, who still has that cost wonât just be uncompetitive, but irrelevant.
One of the most exciting products that Tanjo offers is the Tanjo's Enterprise Brain. What type of machine learning is behind this and what are its use cases?
When we used machine learning to help the US Department of Education create the Learning Registry we saw the power of machine learning to organize and analyze knowledge. When I talk about this I usually show a slide with an image from the last scene of “Raiders of the Lost Ark”; where a clerk is wheeling a drab looking crate through an immense warehouse to file away this incredibly potent artifact that can save or destroy the planet, and it has a little tag on it that says ARC.
What we learned from the Learning Registry project and others is that enterprise search is broken. Companies have untagged and hidden information squirreled away in little data lakes and ponds that are either inaccessible or inscrutable and therefore not transparent to inquiry. In this accelerating Information Age, we are losing knowledge gained every day because of poor storage and retrieval methods.
The Tanjo Enterprise Brain lives inside your firewall, with full source code, connects to everything and has nothing to do but read and scan and organize everything it has access to and wait for the exciting moment when it detects a human trying to do something that could make use of the vast information map at its fingertips. Because it has so much time and power and intimacy with your organizational knowledge, it does not settle for reducing its reading of “War and Peaceâ to #Russiannovel #Tolstoy #warstory #lovestory. Instead it will map it with what we call a “hyperdimensional fingerprint” of up to 4,000 weighted concepts. This seemingly overpowered effort pays great dividends for the research institutions, banks and colleges with Tanjo Enterprise Brains. It is customary for them to realize value far in excess of the license fee just in the organizational knowledge mapping step that is part of training your Enterprise Brain, when leadership learns how much of the knowledge they invest in and depend upon is actually there and what it all means. When the Enterprise Brain is implemented the organization now has a lens with which to see how information entered its systems, who championed it, who challenged it and ultimately how decisions got made. It is becoming a need that is retrospectively obvious. And like the machine learning system JP Morgan implemented, pays dividends forever.
Is there anything else that you would like to share about Tanjo?
Tanjo is working hard right now on a Covid-19 brain. In keeping with the thesis that drives our company, we are determining how to achieve the man and machine balance to make sure the right information and best resources are available to people making important decisions during this crisis. The Tanjo Animated Personas capability will be used to model human population data to track viral spread, but also determine which measure and communication methods and the actual words, will get the behavior we need to help us successfully navigate out of this crisis to a healthier ecosystem for all of us.
This has been a fascinating conversation, readers who may wish to learn more should visit Tanjo.