Artificial Intelligence
Researchers Develop AI Tool to Help Identify Fake News
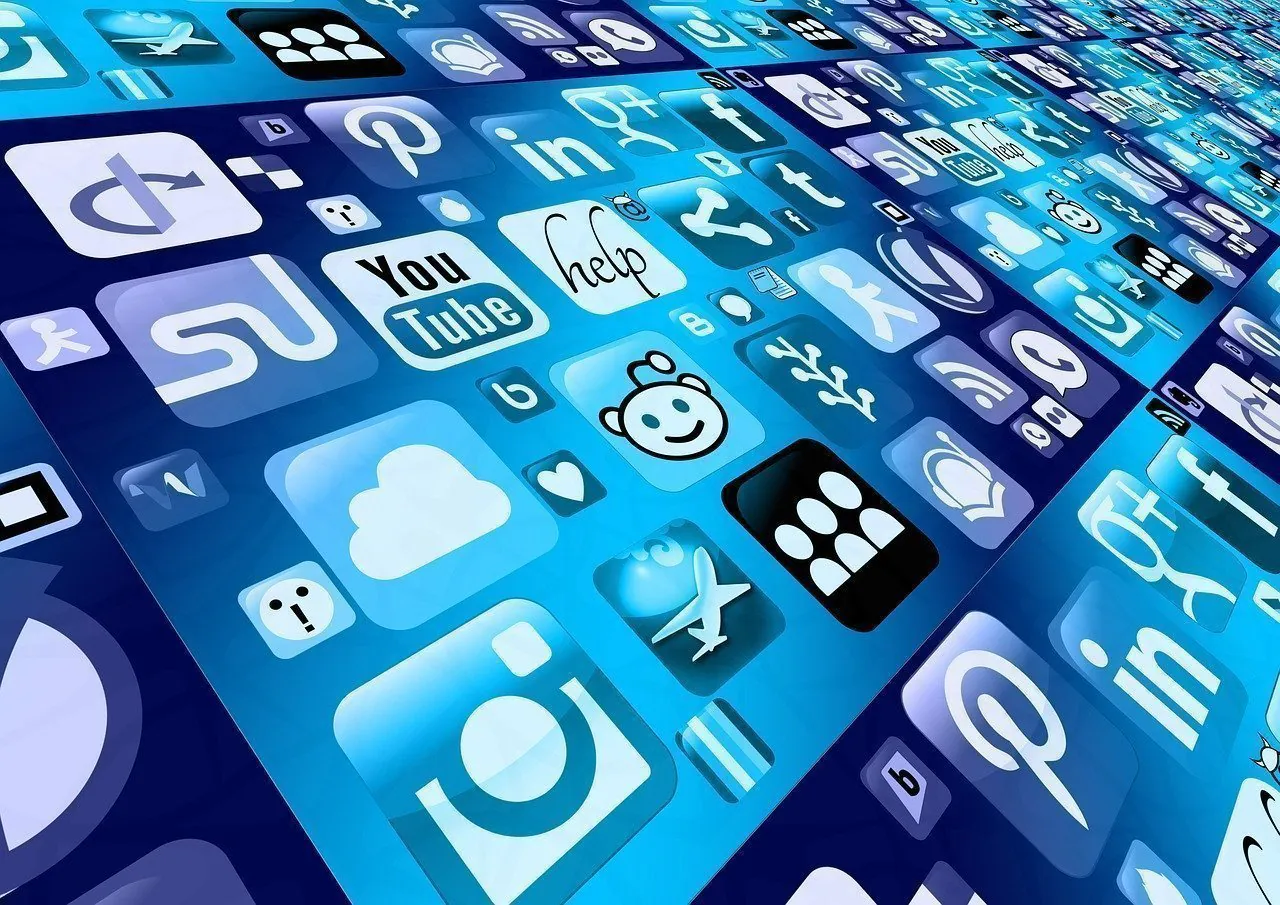
A new artificial intelligence tool has been developed that can help social media networks and news organizations flag false stories.
The tool was developed by researchers at the University of Waterloo. It relies on deep-learning AI algorithms in order to determine if certain claims made in posts and stories hold up. The technology does this by seeing if they are supported by other posts and stories revolving around the same subject.
Alexander Wong is a professor of systems design engineering at Waterloo.
“If they are great, it’s probably a real story,” Wong said. “But if most of the other material isn’t supportive, it’s a strong indication you’re dealing with fake news.”
Researchers decided to develop the tool because of the amount of online posts and news stories that are being revealed as fake or fabricated. News stories are often sent out to deceive or mislead the readers, and they are typically used for political or economic reasons.
The newly developed system is part of an ongoing effort to develop fully-automated technology that is capable of detecting fake news. In one of the key areas of research known as stance detection, the system has a 90 percent accuracy rate.
The system is first given a claim from one post or story and other posts and stories on the same subject. Those are gathered for comparison, and the system is capable of determining whether or not the claim or story is supported or not by the others. It is able to do this nine out of 10 times.
That is now the new benchmark for accuracy, and researchers are using a large dataset that was created for a 2017 scientific competition called the Fake News Challenge.
The technology developed by the Waterloo researchers could become a screening tool used by human fact-checkers. Most social media and news organizations use human fact-checkers, but they often miss certain things that the technology could pick up. This comes at a time when scientists all around the world are working to create a fully automated system for flagging and rooting out fake news.
Wong is a founding member of the Waterloo Artificial Intelligence Institute.
“It augments their capabilities and flags information that doesn’t look quite right for verification,” Wong said. “It Isn't designed to replace people, but to help them fact-check faster and more reliably.”
The AI algorithms that are the foundation of the system were shown tens of thousands of different claims. The claims were shown alongside stories that either supported or did not support them, and the system was eventually able to learn how to determine support or non-support itself. It carried this over to new claim-story pairs that it was shown.
Chris Dulhanty is a graduate student, and he led the project that developed the technology.
“We need to empower journalists to uncover truth and keep us informed,” he said. “This represents one effort in a larger body of work to mitigate the spread of disinformation.”