Interviews
Omri Kohl, CEO & Co-Founder of Pyramid Analytics – Interview Series
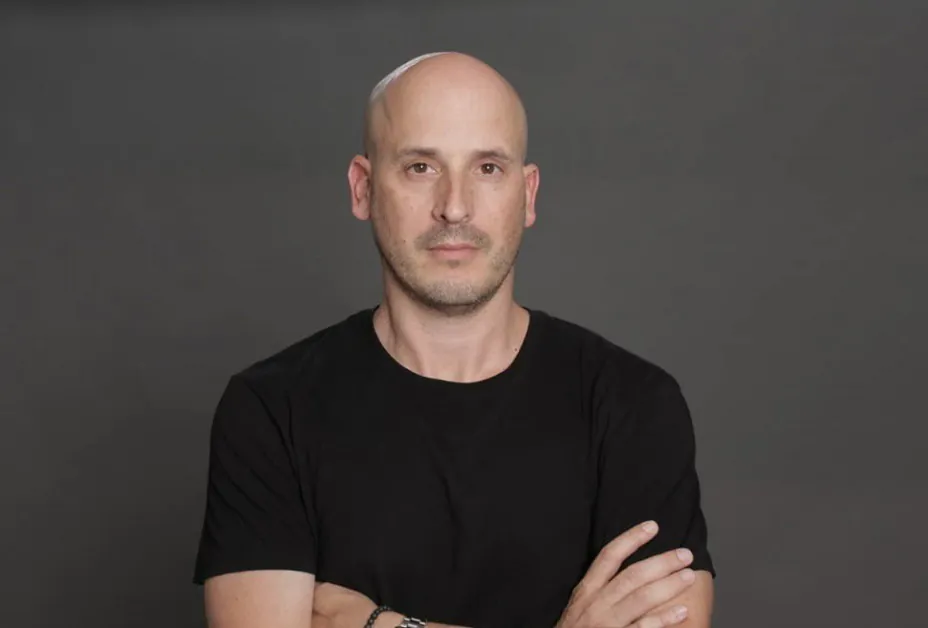
Omri Kohl is the CEO and co-founder of Pyramid Analytics. The Pyramid Decision Intelligence Platform delivers data-driven insights for anyone to make faster, more intelligent decisions. He leads the company’s strategy and operations through a fast-growing data and analytics market. Kohl brings a deep understanding of analytics and AI technologies, valuable management experience, and a natural ability to challenge conventional thinking. Kohl is a highly experienced entrepreneur with a proven track record in developing and managing fast-growth companies. He studied economics, finance, and business management at Bar-Ilan University and has an MBA in International Business Management from New York University, Leonard N. Stern School of Business.
Could you start by explaining what GenBI is, and how it integrates Generative AI with business intelligence to enhance decision-making processes?
GenBI is the framework and mechanics to bring the power of GenAI, LLMs and general AI into analytics, business intelligence and decision making.
Right now, it’s not practical to use GenAI alone to access insights to datasets. It could take over a week to upload enough data to your GenAI tool to get meaningful results. That’s simply not workable, as business data is too dynamic and too sensitive to use in this way. With GenBI, anyone can extract valuable insights from their data, just by asking a question in natural language and seeing the results in the form of a BI dashboard. It takes as little as 30 seconds to receive a relevant, useful answer.
What are the key technological innovations behind GenBI that allow it to understand and execute complex business intelligence tasks through natural language?
Well, without giving away all our secrets, there are essentially three components. First, GenBI prompts LLMs with all the elements they need to produce the correct analytical steps that will produce the requested insight. This is what allows the user to form queries using natural language and even in vague terms, without knowing exactly what type of chart, investigation, or format to request.
Next, the Pyramid Analytics GenBI solution applies these steps to your company’s data, regardless of the specifics of your situation. We’re talking the most basic datasets and simple queries, all the way up to the most sophisticated use cases and complex databases.
Third, Pyramid can carry out these queries on the underlying data and manipulate the results on the fly. An LLM alone can’t produce deep analysis on a database. You need a robot element to find all the necessary information, interpret the user request to produce insights, and pass it on to the BI platform to articulate the results either in plain language or as a dynamic visualization that can later be refined through follow-up queries.
How does GenBI democratize data analytics, particularly for non-technical users?
Quite simply, GenBI allows anyone to tap into the insights they need, regardless of their level of expertise. Traditional BI tools require the user to know which is the best data manipulation technique to receive the necessary results. But most people don’t think in pie charts, scatter charts or tables. They don’t want to have to work out which visualization is the most effective for their situation – they just want answers to their questions.
GenBI delivers these answers to anyone, regardless of their expertise. The user doesn’t need to know all the professional terms or work out if a scattergraph or a pie chart is the best option, and they don’t need to know how to code database queries. They can explore data by using their own words in a natural conversation.
We think of it as the difference between using a paper map to plan your route, and using Google Maps or other navigational app. With a traditional map, you have to work out the best roads to take, think about potential traffic jams, and compare different route possibilities. Today, people just put their destination into the app and hit the road – there’s so much trust in the algorithms that no one questions the suggested route. We’d like to think that GenBI is bringing the same kind of automated magic to corporate datasets.
What has been the feedback from early adopters about the ease of use and learning curve?
We’ve been receiving overwhelmingly positive feedback. The best way we can sum it up is, “Wow!” Users and testers highly appreciate Pyramid’s ease of use, powerful features, and meaningful insights.
Pyramid Analytics has virtually zero learning curve, so there’s nothing holding people back from adopting it on the spot. Approximately three-quarters of all the business teams who’ve tested our solution have adopted it and use it today, because it’s so easy and effective.
Can you share how GenBI has transformed decision-making processes within organizations that have implemented it? Any specific case studies or examples?
Although we’ve been developing it for a long time, we only rolled out GenBI a few weeks ago, so I’m sure you’ll understand that we don’t yet have fully-fledged case studies that we can share, or customer examples that we can name. However, I can tell you that organizations that have thousands of users are suddenly becoming truly data-driven, because everyone can access insights. Users can now unlock the true value of all their data.
GenBI is having a transformative effect on industries like insurance, banking, and finance, as well as retail, manufacturing, and many other verticals. Suddenly, it’s possible for financial advisors, for example, to tap into instant suggestions about the best way to optimize a customer’s portfolio.
What are some of the biggest challenges you faced in developing GenBI, and how did you overcome them?
Pyramid Analytics was already leveraging AI for analytics for many years before we launched the new solution, so most challenges have been ironed out long ago.
The main new element is the addition of a sophisticated query generation technology that works with any LLM to produce accurate results, while keeping data private. We’ve accomplished this by decoupling the data from the query (more on this in a moment).
Another big challenge we had to deal with was that of speed. We’re talking about the Google era, where people expect answers now, not in an hour or even half an hour. We made sure to speed up processing and optimize all workflows to reduce friction.
Then there’s the need to prevent hallucination. Chatbots are prone to hallucinations which skew results and undermine reliability. We’ve worked hard to avoid those while still maintaining dynamic results.
How do you handle issues related to data security and privacy?
That’s a great question, because data privacy and security is the biggest obstacle to successful GenAI analytics. Everyone is – quite rightly – concerned about the idea of exposing highly sensitive corporate data to third-party AI engines, but they also want the language interpretation capabilities and data insights that these engines can deliver.
That’s why we never share actual data with the LLMs we work with. Pyramid flips the entire premise on its head by serving as an intermediary between your company’s information and the LLM. We allow you to submit the request, and then we hand it to the LLM along with descriptions of what we call the “ingredients,” basically just the metadata.
The LLM then returns a “recipe,” which explains how to turn the user’s question into a data analytics prompt. Then Pyramid runs that recipe on the data that you’ve already connected securely on your self-hosted install, so that no data ever reaches the LLM. We mash up the results to serve them back to you in an easily understandable, visual format. Essentially, nothing that could compromise your security and privacy gets exposed or leaves the safety of your organization’s firewall.
For organizations looking to integrate GenBI into their existing data infrastructures, what does the implementation process look like? Are there any prerequisites or preparations needed?
The implementation process for Pyramid Analytics couldn’t be easier or faster. Users need very few prerequisites and preparations, and you can get the whole thing up and running in under an hour. You don’t need to move data into a new framework or change anything about your data strategy, because Pyramid queries your data directly where it resides.
There’s also no need to explain your data to the solution, or to define columns. It’s as simple as uploading a CSV dataset or connecting your SQL database. The same goes for any relational database of any sort. It takes only a few minutes to connect your data, and then you can ask your first question seconds later.
That said, you can tweak the structure if you want, like changing the joining model or redefining columns. It does take some time and effort, but we’re talking minutes, not a months-long dev project. Our customers are often shocked that Pyramid is up and running on their classic data warehouse or data lake within five minutes or so.
You also don’t need to come up with very specific, accurate, or even intelligent questions to get powerful results. You can make spelling mistakes and use incorrect phrasing, and Pyramid will unravel them and produce a meaningful and valuable answer. What you do need is some knowledge about the data you’re asking about.
Looking ahead, what's your strategic vision for Pyramid Analytics over the next five years? How do you see your solutions evolving to meet changing market demands?
The next big frontier is supporting scalable, highly specific queries. Users are eager to be able to ask very precise questions, such as questions about personalized entities, and LLMs can’t yet produce intelligent answers in these cases, because they don’t have that kind of detailed insight into the specifics of your database.
We’re facing the challenge of how to use language models to ask about the specifics of your data without instantly connecting your entire, gigantic data lake to the LLM. How do you finetune your LLM about data that gets rehydrated every two seconds? We can manage this for fixed points like countries, locations, and even dates, but not for something idiosyncratic like names, even though we are very close to it today.
Another challenge is for users to be able to ask their own mathematical interpretations of the data, applying their own formulae. It’s difficult not because the formula is hard to enact, but because understanding what the user wants and getting the correct syntax is challenging. We’re working on solving both these challenges, and when we do, we’ll have passed the next eureka point.
Thank you for the great interview, readers who wish to learn more should visit Pyramid Analytics.