Thought Leaders
Institutional Investors are Looking For Returns. Machine Overlays Can Help Find Them
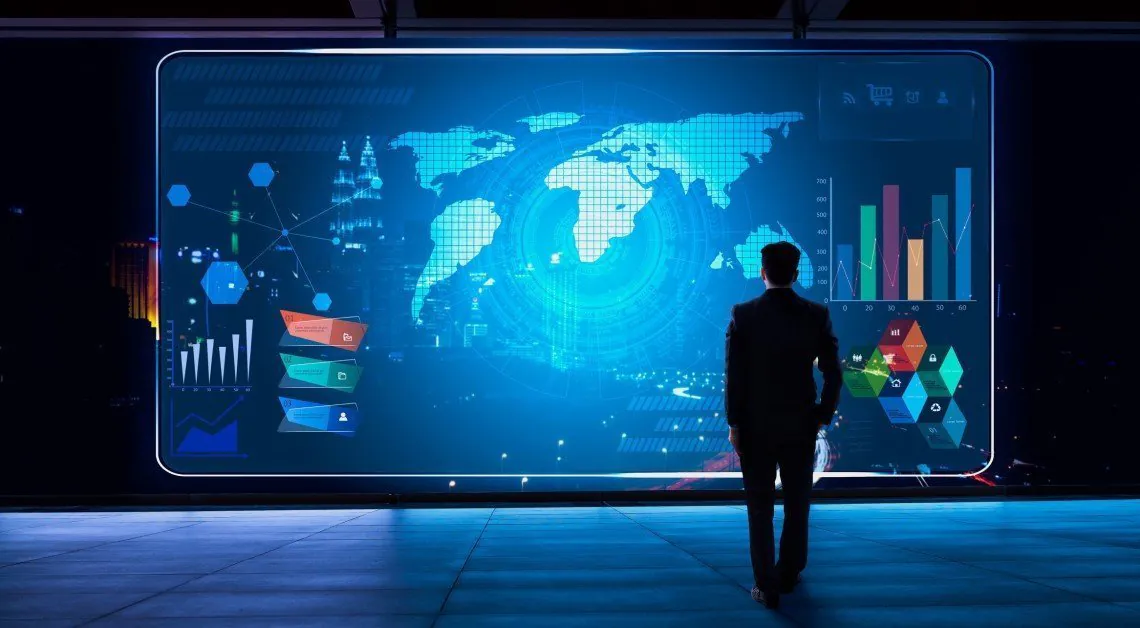
By Nicholas Abe, co-founder & COO of Boosted.ai
How can investors get the best of both worlds from quantitative and fundamental approaches? By implementing machine learning overlays, writes Nick Abe, co-founder and Chief Operating Officer of Boosted.ai. Fundamental managers are leaving gains on the table by not adapting to changing technologies and institutional investor demand. Abe demonstrates that combining their financial domain expertise with cutting-edge artificial intelligence tools can increase alpha and Sharpe.
Both sides of the investing spectrum – quantitative and fundamental – have had issues lately. Even the most sophisticated investors struggled in 2020 due to the unforeseen volatility that the COVID-19 pandemic brought to market.
The quantitative approach has been slowly building within large asset managers as they create their own quant teams. However, the promise of having an edge from modern technology has been met with the difficulties of putting successful machine learning into practice, largely due to the expertise required and the high cost of developing a functioning program.
Successful quantitative shops employ swaths of Ph.D.’s, data scientists and engineers to make sense of large amounts of complex data – and even then sometimes fail. Finding predictive power from data is hard, and black swan events like COVID-19 and other regime shifts can render that data obsolete without human oversight.
Fundamental Failings
Most people are aware of the principles of fundamental analysis – studying financial statements and incorporating economic factors to make decisions on where investors should invest for the best returns given goals and risk appetite. Investors have practiced and honed this time-consuming approach to delivering returns for decades. Some, however, are warming to taking advantage of modern technology like machine learning and alternative data to sharpen performance, synthesize information in less time and curtail any cognitive biases that may interfere in the decision making process.
Further, fundamental active investment management is facing immense challenges, ranging from fee compression and technological advancements to shifting investor sentiment towards low-cost ETFs.
What do both quantitative and fundamental approaches have in common? They study the world around them to make informed decisions about where best to deploy capital for returns.
But what if there were a third option?
The Call for Machine Learning in Fundamental Management
Machine learning has revolutionized industries and day-to-day life. From Google Translate to self-driving cars, technology is transforming the world much like the industrial revolution before it, and the investment management industry will not be immune to the changes. According to a 2019 study by the CFA Institute that surveyed portfolio managers, only 10% of portfolio managers had used any artificial intelligence or machine learning in their investment process.
As technology continues to progress, machine learning techniques will become a non-negotiable aspect of investment management. However, many machine learning applications require programming knowledge foreign to traditional managers who are more confident in their own fundamental analysis, which they can do on their own and by default have a deeper understanding of.
Given the roadblocks above, how can fundamental managers successfully adapt?
Combining For a Better Process: Machine Learning Overlays
Adding a machine learning overlay to a portfolio is just one example of a marriage of the fundamental investment manager’s expertise and the technological advantages AI has to offer.
Machine overlays solve for the roadblocks of fundamental investors looking to incorporate the technology. They are easy-to-use and can be deployed on top of traditional investors' existing portfolios without any programming knowledge required. They provide full explainability of the machine’s reasoning, showcasing which variables the machine learning viewed as important in its decision making. This helps fundamental managers feel more comfortable implementing the intelligence in their process.
For example, a Boosted Insights machine learning overlay takes an investment manager’s existing portfolio and adjusts the stock position weightings slightly. It does not add any new positions – rather, it adjusts the weightings (long or short) of stocks in the manager’s existing portfolio. Based on its findings, stocks that rank highly may have their weightings increased and stocks that rank poorly may have their weightings reduced.
In the end, machine learning overlays allow a fundamental investment manager to combine their stock-picking acumen with cutting edge, finance specific AI/ML for better results.
An investment manager may like Facebook, Apple, Amazon, Netflix and Google (FAANG) stocks and find that they offer good performance in their portfolio but have all five at equal weights. The addition of the Boosted Insights machine learning overlay allows the machine to alter the weightings slightly – say, Facebook gets reduced to 18.5% and Apple gets increased to 21.5%. Those minor differences, while keeping the investment manager’s portfolio the exact same, can result in better outcomes in terms of return, alpha, and volatility.
We have found that these types of models are able to improve portfolios that had high alpha already by only adjusting the weightings of the stocks and not having to adjust the composition. Beta remained consistent as the baseline allocations were adjusted by the model overlays.
Machine learning for better investing
Machine learning has and will continue to disrupt industries. Investment managers can enhance their portfolio objectives by implementing machine learning into their process, but in a way that is complementary and organic to their workflow. A good way to dip their toes into the waters of machine learning techniques is to implement a machine learning overlay.
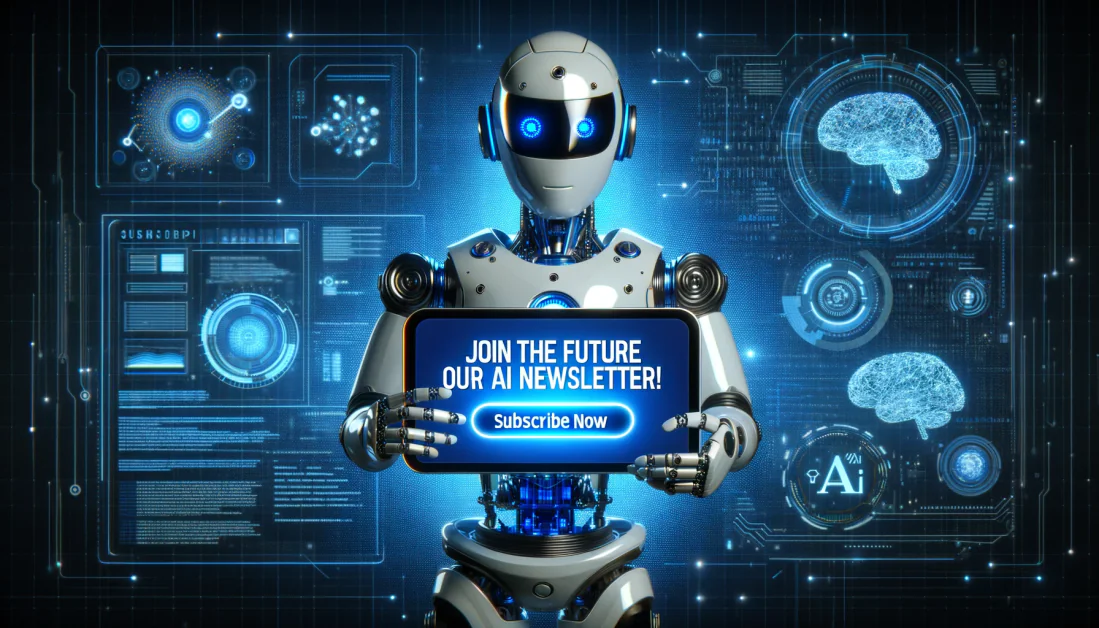
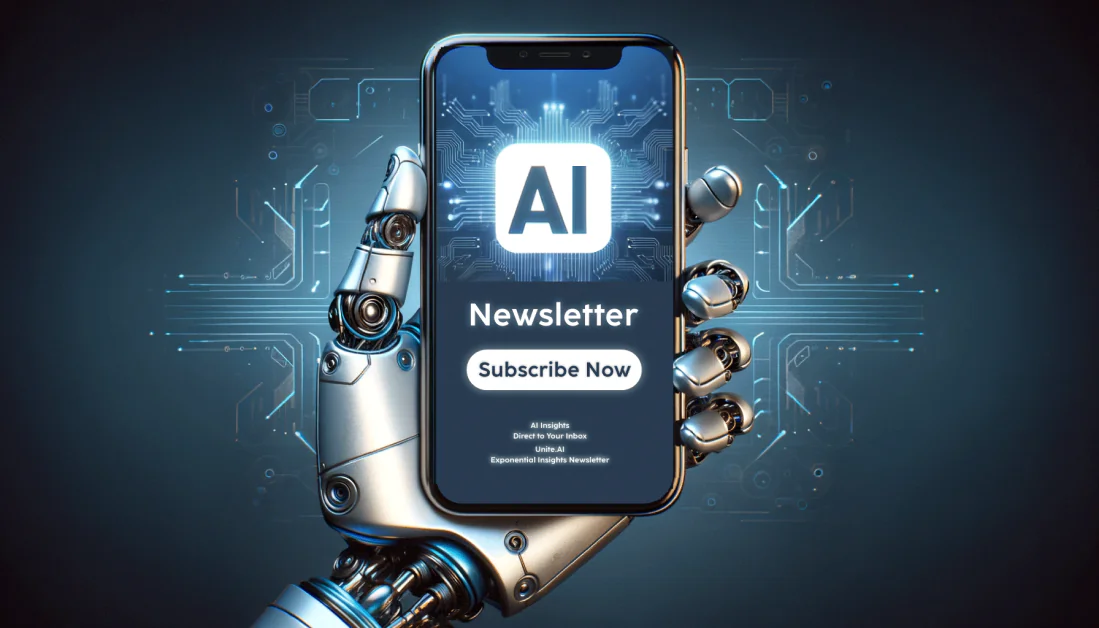