Interviews
Gil Elbaz, Co-founder & CTO of Datagen – Interview Series
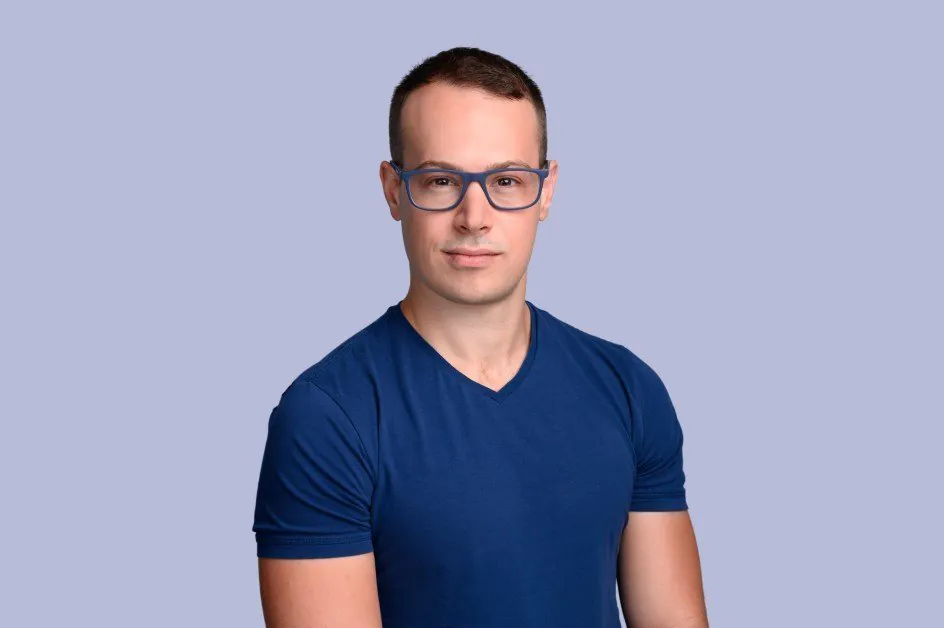
Gil Elbaz is Datagen’s CTO and Co-founder, based in Tel Aviv. He received his B.Sc and M.Sc from the Technion. Gil’s thesis research was focused on 3D Computer Vision and has been published at CVPR, the top computer vision research conference in the world. Datagen is a pioneer in the new field of Simulated Data, a subset of synthetic data, which concentrates on photo-realistically recreating the world around us. The company launched from stealth with over $18M in funding in March 2021 and is now working with a number of Fortune 100 companies in augmented/virtual reality, robotics, and automotive, including the majority of the top U.S. tech giants.
What initially attracted you to robotics and machine learning?
Sci-Fi books, like Isaac Asimov's Foundation Series and iRobot always got me thinking about a future in which robots were an integral part of our day-to-day lives. There are so many boring, repetitive tasks that people do; I knew that I didn’t want to do them, and I couldn’t imagine anyone else wanting to. Considering robotics is a technological inevitability, I thought that going in that direction would be a smart, “future-proof” career decision.
So, I initially approached the field focusing on the physical aspects of the subject, and got my degree in Mechanical Engineering from Technion in Haifa, Israel. Towards the end of my degree, I started diving deep into the world of CAD tools and capabilities. These are the tools which allow mechanical engineers to design structures and mechanical devices (anything from a bridge to a car). I saw an enormous opportunity to make a large impact without dealing with the slow iterations of the physical world. In practice, these programs had very little, if any, machine learning / computer vision capabilities integrated, that helped engineers create simpler, cheaper and more stable mechanical systems (this is back in 2015). I set off in the direction of Computer Vision on 3D data with deep learning (very new back then) with the goal of making smarter CAD programs. Working in the early days of modern deep learning, felt like being in the part of something which could be really big — similar to the internet.
In practice, my research was the first to bring the Deep Learning revolution to our faculty at the Technion. This later turned into a paper accepted into the top Computer Vision conference in the world, CVPR, and I flew to Hawaii at CVPR 2017. Presenting my paper and meeting the people really opened my eyes to the scale of the computer vision community (which today is at least 10x larger), thousands of participants all passionately working on research in the field. That event pretty much cemented my direction, showing me the power of computer vision and the potential waiting to be unlocked.
Could you share the genesis story behind Datagen?
Datagen was founded in 2018 with a mission to transform how teams get their data for computer vision network training. The year before, we saw a demo of the Oculus Rift, which consisted of a VR headset and a handheld remote control device. After the demo, we found ourselves wondering, “with sophisticated cameras embedded in the headset, why was a handheld device needed to connect the virtual space to physical space (i.e. track hand movement)?” The neural networks were already sophisticated enough to handle it, so what was the problem?” And that’s when the light bulb went off — Data! We immediately saw the huge opportunity to solve 3D spatial presence challenges using advanced computer vision and 3D metadata. Rather than focusing solely on VR/AR, we took a more holistic approach, concentrating on the seemingly intractable problem of generating sufficient (and accurate) training data to enable real-world 3D AI applications.
With a focus on humans and human-environment interaction, Datagen is a pioneer in the new field of Simulated Data, a subset of synthetic data, which concentrates on photo-realistically recreating the world around us. Today, we work with the most innovative companies in the world to fuel and accelerate their computer vision development and are backed by some of the most respected investors in the space.
For readers who are unfamiliar could you explain what specifically is synthetic data?
Synthetic data is any training data that – instead of being gathered via direct measurement or observation of the real world – is generated either algorithmically or via simulation. In the context of computer vision, synthetic data is computer-generated images with associated metadata needed for training artificial intelligences. With privacy issues, and very real physical and economic limitations to real-world image data, it’s hard to overstate the significance of synthetic data to machine learning and AI. In a recent report, Gartner predicted that, by 2024, most of the data used in the field of AI will be artificially generated for those reasons.
What are some benefits of synthetic data compared to manual data acquisition?
The short answer is, think of every aspect of manual data acquisition that’s undesirable and remove them from the process — those are the benefits of synthetic data.
Generating diverse datasets at scale for computer vision training is a costly, time-consuming process, and variance is very limited by the mere fact that situating humans in specific locations and photographing them is a complicated process — far more complicated and costly than doing so in a simulated environment. Another major benefit is effectively eliminating the need for manual annotation, which is tedious, time-consuming, and prone to human error.
Datagen refers to simulated data as a subset of synthetic data. Could you elaborate on what simulated data is?
Simulated data is synthetic data that is generated through simulation. We use GANs (as well as some other cutting-edge machine learning methods) to generate 3-D objects and place them within highly realistic 3-D simulations of the real world. What that looks like is a first-person “virtual picture-taking” process, but operating within a photo-realistic, physics-based system. These simulations produce visual data (as if it was gathered in the real world), together with a full range of annotations (physics, lighting, etc.). So, Simulated Data is synthetic data that is photo-realistic, contextually generated, 3-D imagery, gathered in a simulated environment.
How does Datagen generate tailored simulated data?
Datagen’s technology generates simulated data that’s both readily scalable and hand-tailored to address the unique needs of each customer’s distinct application. We do so by taking into account every aspect of every project — from the computer vision system being employed to the demographic makeup of the region in which it will be operating. Whether working directly with our customers, or simply enabling their own engineers, the Datagen process begins with establishing key parameters for each specific use case, such as lens specifications, lighting, environment, demographic distribution, and so on. Datagen uses GANs and other cutting-edge tools and techniques to generate an immense variety of assets, including everything from human heads with dynamic facial expressions to train AI in emotional analysis, to vehicle interiors for in-cabin passenger monitoring, and home environments for video conferencing applications, just to name a few. For each asset type, Datagen introduces variance across countless discrete axes (from skin tone and brow height, to the size, color, and shape of household furniture), using parameters that are finely-tuned to reflect the specific application at hand.
Thanks to these capabilities, Datagen’s datasets are not only large and highly-varied, but optimized for the purposes of training a unique system to perform a unique task (or set of tasks) in the unique environment or setting in which it will be employed — all without compromising the capacity to scale. We also take into account the specific annotation/metadata requirements of each application.
What are some examples of solutions in robotics where synthetic and/or simulated data is used?
One of the greatest advantages of using simulated data in robotics is the ability to generate images of hardware that’s still in development. This way, your robot’s brain (AI) and body (hardware) can be developed side-by-side. Now, the training can evolve as the specifications evolve, rather than waiting until your final product is fully prototyped before you can take photos of it and begin developing the AI.
Also, because simulated data is generated in-context, you can account for interaction between your robot and its environment much more easily. So, if you imagine a robot that grabs and removes defective products from an assembly line, simulated data would allow you to not only generate data for every physical defect imaginable in the product, but also from the robot’s perspective to capture the robotic arm’s full range of motion, its interaction with the object it is grabbing. What’s more, 3d metadata means there’s no need to painstakingly annotate image after image to ensure the robot can properly identify the product, the defects, its arm, or anything else in its field of view.
What are some use cases for using simulated data in smart cars?
Simulated data in smart car development makes it infinitely easier to develop datasets for specific car models as they’re being designed, iterating in concert with the car itself as it progresses through the various phases of design and production. With simulated image data, engineers can also use in-cabin vision more effectively to identify drowsy or distracted drivers, if a driver has taken their hand off the wheel, or any number of edge cases to account for driver safety. It also enables engineers to account for greater diversity in the drivers and passengers, and introduce variance in the form of image angle and lighting — all without infringing on the privacy of real people.
Recently, Datagen announced a large number of exciting new hires, what does this mean for the future of the company?
The recent additions to our advisory board and executive leadership include some of the most brilliant, accomplished professionals in the field of AI and Computer Vision. Their knowledge, insight, and experience will help orient and accelerate Datagen’s growth as we navigate an industry that’s still young and brimming with opportunity. In a field with so many unknowns, nothing’s more valuable than knowledge.
Is there anything else that you would like to share about Datagen?
Based out of Tel Aviv, Datagen is part of a much larger economic and cultural shift that has taken place in Israel, and we’re proud to be a part of it. In a short period of time, Israel (Tel Aviv in particular), has grown into a major global tech hub, with a thriving startup ecosystem and an energetic investment community. Though Israel is often considered a cyber-security centered tech hub, AI and data-centric tech has grown exponentially in recent years here. Today, there are more than 680 artificial intelligence companies in Israel, which have collectively raised $4.5Bl. This growth explosion over the last few years is due largely in part to the high concentration of engineers and Israel’s world-renowned universities. These academic institutions provide access to talent and cutting-edge new technology development in the space. In the last two months, Datagen has hired more than 20 employees and plans to bring on additional team members across sales and marketing, software and DevOps, and product departments.
Thank you for the great interview, readers who wish to learn more should visit Datagen.