Interviews
Eric Landau, Co-Founder & CEO of Encord – Interview Series
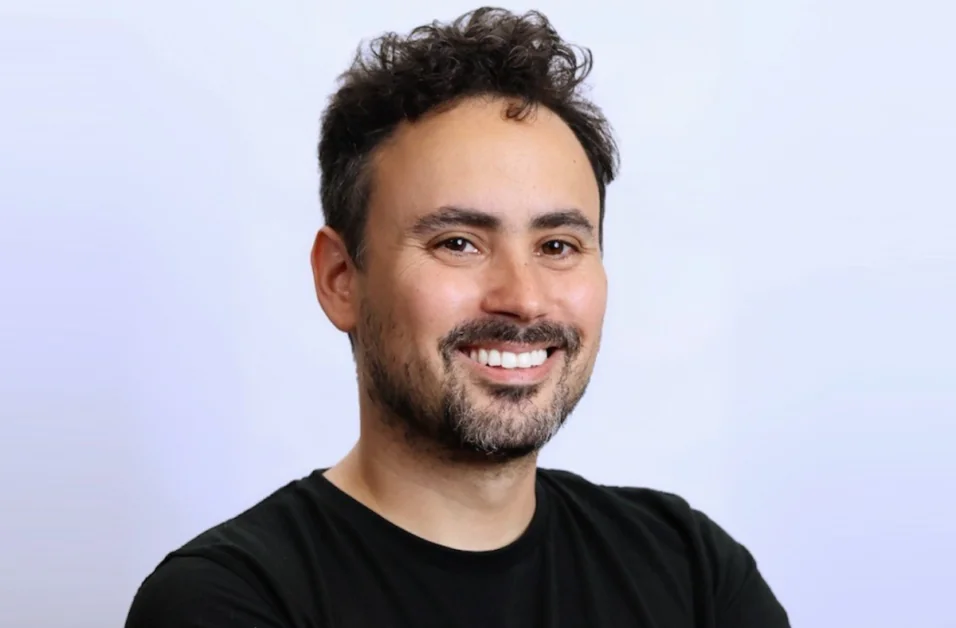
Eric Landau is the CEO & Co-Founder of Encord, an active learning platform for computer vision. Eric was the lead quantitative researcher on a global equity delta-one desk, putting thousands of models into production. Before Encord, he spent nearly a decade in high-frequency trading at DRW. He holds an S.M. in Applied Physics from Harvard University, M.S. in Electrical Engineering, and B.S. in Physics from Stanford University.
In his spare time, Eric enjoys playing with ChatGPT and large language models and craft cocktail making.
What inspired you to co-found Encord, and how did your experience in particle physics and quantitative finance shape your approach to solving the “data problem” in AI?
I first started thinking about machine learning while working in particle physics and dealing with very large datasets during my time at the Stanford Linear Accelerator Center (SLAC). I was using software designed for physicists by physicists, which is to say there was a lot to be desired in terms of a pleasant user experience. With easier tools, I would have been able to run analyses much faster.
Later, working in quantitative finance at DRW, I was responsible for creating thousands of models that were deployed into production. Similar to my experience in physics, I found that high-quality data was critical in making accurate models and that managing complex, large-scale data is difficult. Ulrik had a similar experience visualizing large image datasets for computer vision.
When I heard about his initial idea for Encord, I was immediately on board and understood the importance. Together, Ulrik and I saw a huge opportunity to build a platform to automate and streamline the AI data development process, making it easier for teams to get the best data into models and build trustworthy AI systems.
Can you elaborate on the vision behind Encord and how it compares to the early days of computing or the internet in terms of potential and challenges?
Encord's vision is to be the foundational platform that enterprises rely on to transform their data into functional AI models. We are the layer between a company’s data and their AI.
In many ways, AI mirrors previous paradigm shifts like personal computing and the Internet in that it will become integral to workflows for every individual, business, nation, and industry. Unlike previous technological revolutions, which have been largely bottlenecked by Moore’s law of compounded computational growth of 30x every 10 years, AI development has benefited from simultaneous innovations. It is thus moving at a much faster pace. In the words of NVIDIA’s Jensen Huang: “For the very first time, we are seeing compounded exponentials…We are compounding at a million times every ten years. Not a hundred times, not a thousand times, a million times.” Without hyperbole, we are witnessing the fastest-moving technology in human history.
The potential here is vast: by automating and scaling the management of high-quality data for AI, we’re addressing a bottleneck preventing broader AI adoption. The challenges are reminiscent of early-day hurdles in previous technological eras: silos, lack of best practices, limitations for non-technical users, and a shortage of well-defined abstractions.
Encord Index is positioned as a key tool for managing and curating AI data. How does it differentiate itself from other data management platforms currently available?
There are a few ways that Encord Index stands out:
Index is scalable: Allows users to manage billions, not millions, of data points. Other tools face scalability issues for unstructured data and are limited in consolidating all relevant data in an organization.
Index is flexible: Integrates directly with private data storage and cloud storage providers such as AWS, GCP, and Azure. Unlike other tools that are limited to a single cloud provider or internal storage system, Index is agnostic to where the data is located. It lets you manage data from many sources with appropriate governance and access controls that allow them to develop secure and compliant AI applications.
Index is multimodal: Supports multimodal AI, managing data in the form of images, videos, audio, text, documents and more. Index is not limited to a single form of data like many LLM tools today. Human cognition is multimodal, and we believe multimodal AI will be at the heart of the next wave of AI advancements, which will supplant chatbots and LLMs.
In what ways does Encord Index enhance the process of selecting the right data for AI models, and what impact does this have on model performance?
Encord Index enhances data selection by automating the curation of large datasets, helping teams identify and retain only the most relevant data while removing uninformative or biased data. This process not only reduces the size of datasets but also significantly improves the quality of the data used for training AI models. Our customers have seen up to a 20% improvement in their models while achieving a 35% reduction in dataset size and saving hundreds of thousands of dollars in compute and human annotation costs.
With the rapid integration of cutting-edge technologies like Meta’s Segment Anything Model, how does Encord stay ahead in the fast-evolving AI landscape?
We intentionally built the platform to be able to adapt to new technologies quickly. We focus on providing a scalable, software-first approach that easily incorporates advancements like SAM, ensuring that our users are always equipped with the latest tools to stay competitive.
We plan to stay ahead by focusing on multimodal AI. The Encord platform can already manage complex data types such as images, videos, and text, so as more advancements in multimodal AI come our way, we’re ready.
What are the most common challenges companies face when managing AI data, and how does Encord help address these?
There are 3 main challenges companies face:
- Poor data organization and controls: As enterprises prepare to implement AI solutions, they are often met with the reality of siloed and unorganized data that is not AI-ready. This data often lacks strong governance around it, limiting much of it from being used in AI systems.
- Lack of human experts: As AI models tackle increasingly complex problems, there will soon be a shortage of human domain experts to prepare and validate data. As a company’s AI demands increase, scaling that human workforce is challenging and costly.
- Unscalable tooling: Performant AI models are very data-hungry in terms of data needed for fine-tuning, validation, RAG, and other workflows. The previous generation of tools is not equipped to manage the amount of data and types of data required for today’s production-grade models.
Encord fixes these problems by automating the process of curating data at scale, making it easy to identify impactful data from problematic data and ensuring the creation of effective training and validation datasets. It uses a software-first approach that is easy to scale up or down as data management needs change. Our AI-assisted annotation tools empower human-in-the-loop domain experts to maximize workflow efficiency. This process is particularly crucial in industries such as financial services and healthcare, where AI trainers are costly. We make it easy to manage and understand all of an organization’s unstructured data, reducing the need for manual labor.
How does Encord tackle the issue of data bias and under-represented areas within datasets to ensure fair and balanced AI models?
Tackling data bias is a critical focus for us at Encord. Our platform automatically identifies and surfaces areas where data might be biased, allowing AI teams to address these issues before they impact model performance. We also ensure that under-represented areas within datasets are properly included, which helps in developing fairer and more balanced AI models. By using our curation tools, teams can be confident that their models are trained on diverse and representative data.
Encord recently secured $30 million in Series B funding. How will this funding accelerate your product roadmap and expansion plans?
The $30 million in Series B funding will be used to drastically increase the size of our product, engineering, and AI research teams over the next six months and accelerate the development of Encord Index and other new features. We’re also expanding our presence in San Francisco with a new office, and this funding will help us scale our operations to support our growing customer base.
As the youngest AI company from Y Combinator to raise a Series B, what do you attribute to Encord's rapid growth and success?
One of the reasons we have been able to grow quickly is that we have adopted an extremely customer-centric focus in all areas of the company. We are constantly communicating with customers, listening closely to their problems, and “bear hugging” them to get to solutions. By hyper-focusing on customer needs rather than hype, we’ve created a platform that resonates with top AI teams across various industries. Our customers have been instrumental in getting us to where we are today. Our ability to scale quickly and effectively manage the complexity of AI data has made us an attractive solution for enterprises.
We also owe much of our success to our teammates, partners, and investors, who have all worked tirelessly to champion Encord. Working with world-class product, engineering, and go-to-market teams has been enormously impactful in our growth.
Given the increasing importance of data in AI, how do you see the role of AI data platforms like Encord evolving in the next five years?
As AI applications grow in complexity, the need for efficient and scalable data management solutions will only increase. I believe that every enterprise will eventually have an AI department, much like how IT departments exist today. Encord will be the only platform they need to manage the vast amounts of data required for AI and get models to production quickly.
Thank you for the great interview, readers who wish to learn more should visit Encord.