Artificial Intelligence
AI Hardware Technology Imitates Changes in Neural Network Topology
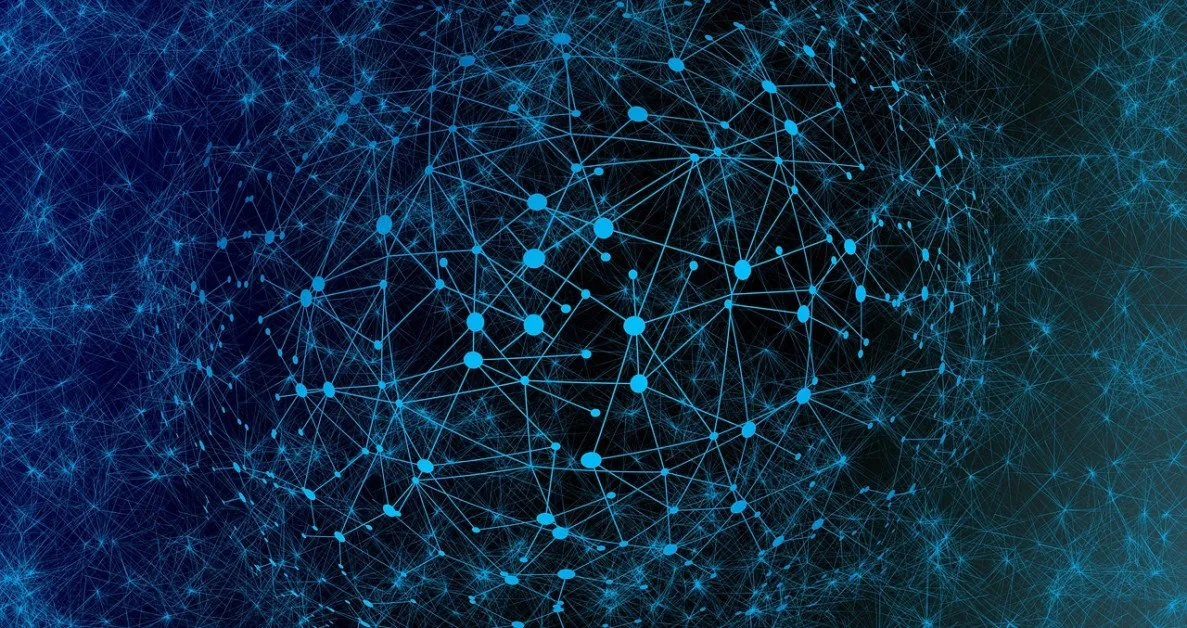
A group of researchers at The Korea Advanced Institute of Science and Technology (KAIST) has proposed a new system inspired by the neuromodulation of the brain, which is called a “stashing system.” This newly proposed system requires less energy consumption.
The team was led by Professor Kyung Min Kim from the Department of Materials Science and Engineering. The research was published in Advanced Functional Materials and supported by KAIST, the National Research Foundation of Korea, the National NanoFab Center, and SK Hynix.
Imitating Neural Network Topology
The researchers developed a technology that can efficiently handle mathematical operations for artificial intelligence by imitating the changes in the topology of the neural network depending on the situation. This was inspired by the human brain, which can change its neural topology in real time, enabling it to learn to store or recall memories when needed.
This new type of AI learning method directly implements neural coordination circuit configurations.
In order for the effective implementation of AI in electronic devices, it is important for customized hardware development to be supported. With that said, most electronic devices created for AI require high power consumption. If they are to carry out large-scale tasks, they also need highly integrated memory arrays. These limitations in consumption and integration have proven hard to overcome, so researchers have begun to look deeper inside the human brain to know how it solves problems.
Highly Efficient Technology
The team demonstrated the efficiency of the new technology by creating artificial neural network hardware with a self-rectifying synaptic array and algorithm referred to as a “stashing system.” This hardware was developed to conduct AI learning, and it was able to reduce energy by 37% within the stashing system without suffering accuracy degradation.
“In this study, we implemented the learning method of the human brain with only a simple circuit composition and through this we were able to reduce the energy needed by nearly 40 percent,” Professor Kim said.
One of the important aspects of this new stashing system mimicking the brain’s activity is that it’s compatible with existing electronic devices and commercialized semiconductor hardware. The system could play a big role in the design of next-generation semiconductor chips for AI.
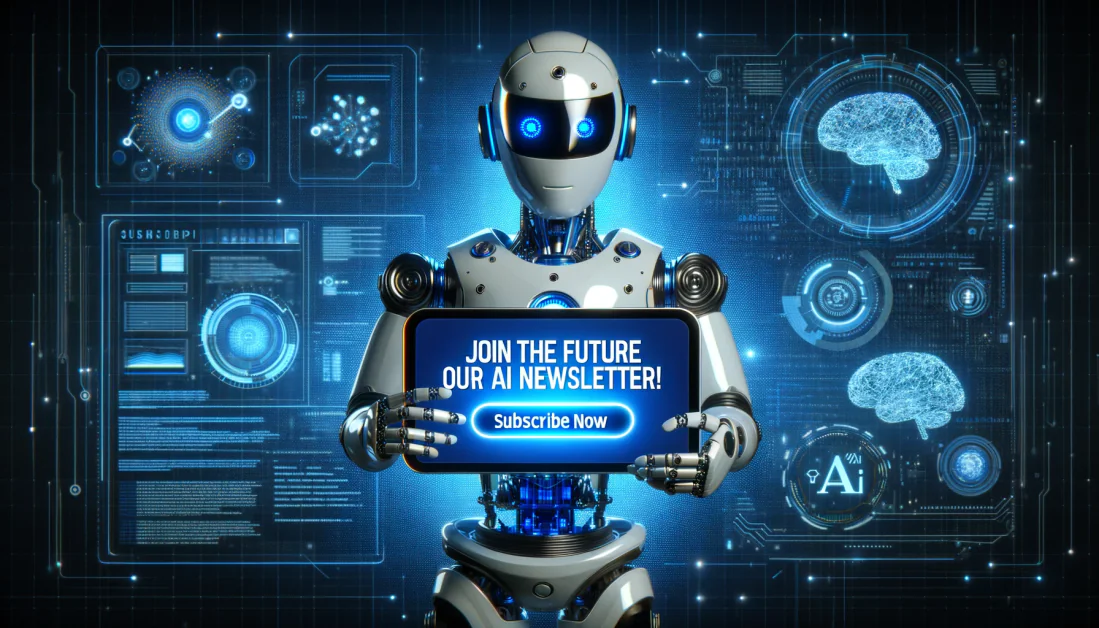
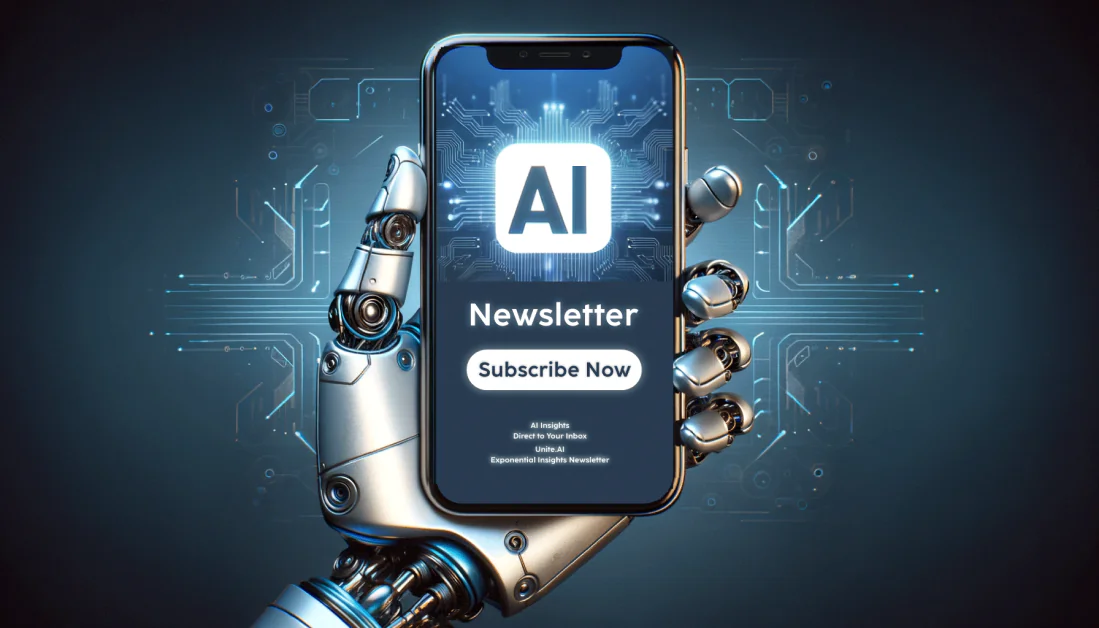